Machine learning sto的問題,透過圖書和論文來找解法和答案更準確安心。 我們找到下列股價、配息、目標價等股票新聞資訊
Machine learning sto的問題,我們搜遍了碩博士論文和台灣出版的書籍,推薦Costa, Rui寫的 Programming Google Cloud: Building Cloud Native Applications with Gcp 和Albert, Mark/ Petrov, Plamen/ Ronanki, Rajeev的 Applied Heath Care Analytics: Enabling Transformative Health Care Through Data Science, Machine Learning, and Cognitive Computin都 可以從中找到所需的評價。
另外網站Leveraging Machine Learning for Fraud Prevention | PayPal US也說明:PayPal uses machine learning models and comprehensive data sets to help merchants detect and prevent fraud. Learn about our approach to ...
這兩本書分別來自 和所出版 。
國立虎尾科技大學 飛機工程系航空與電子科技碩士班 楊世英所指導 陳柏諺的 穿音速流通過機翼釋放MK-82彈體之高度與前彈射力變化數值探討 (2021),提出Machine learning sto關鍵因素是什麼,來自於穿音速、高度、彈射力。
而第二篇論文國立臺灣師範大學 工業教育學系 蘇友珊所指導 蔡志成的 醫療影像辨識新興技術預測-以專利分析法探討 (2020),提出因為有 智慧醫療、影像辨識技術、費雪成長模型、羅吉斯成長模型、生命週期的重點而找出了 Machine learning sto的解答。
最後網站STOP Learning Machine Learning - YouTube則補充:Focus on the SKILLS you need right now!!! If you're interested in the fastest path to a career in machine learning or any other data role my ...
除了Machine learning sto,大家也想知道這些:
Programming Google Cloud: Building Cloud Native Applications with Gcp
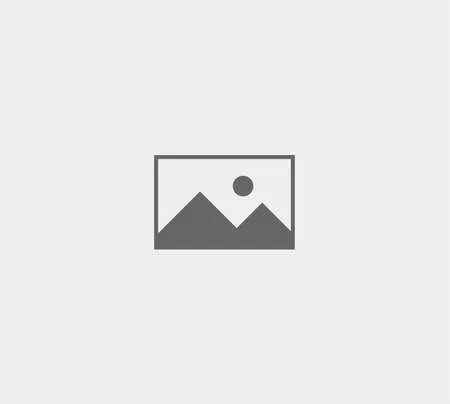
為了解決Machine learning sto 的問題,作者Costa, Rui 這樣論述:
Companies looking to move enterprise applications to the cloud are busy weighing several options, such as the use of containers, machine learning, and serverless computing. There’s a better way. Instead of helping you fit your use case to individual technologies, this practical guide explains how
to use these technologies to fit your use case. Author Rui Costa, a learning consultant with Google, demonstrates this approach by showing you how to run your application on Google Cloud. Each chapter is dedicated to an area of technology that you need to address when planning and deploying your a
pplication. This book starts by presenting a detailed fictional use case, followed by chapters that focus on the building blocks necessary to deploy a secure enterprise application successfully. Build serverless applications with Google Cloud Functions Explore use cases for deploying a real-time mes
saging service Deploy applications to Google Kubernetes Engine (GKE) Build multiregional GKE clusters Integrate continuous integration and continuous delivery with your application Incorporate Google Cloud APIs, including speech-to-text and data loss prevention Enrich data with Google Cloud Dataflow
Secure your application with Google Cloud Identity-Aware Proxy Explore BigQuery and visualization with Looker and BigQuery SDKs
穿音速流通過機翼釋放MK-82彈體之高度與前彈射力變化數值探討
為了解決Machine learning sto 的問題,作者陳柏諺 這樣論述:
本文使用商用軟體ESI CFD來研究穿音速流通過機翼釋放MK-82彈體之高度與前彈射力變化的數值分析,在卡式座標系統下求解三維非穩態Euler方程式和6-DOF剛體運動方程式,使用Chimera網格系統,讓不同區塊網格流場資料各自計算,而重疊區的格點資訊互相傳遞。將機翼、MK-82彈體兩者流場耦合並分析6-DOF剛體運動方程式獲得計算結果,其彈體重心、翻滾角計算之結果與實驗值比對後趨勢接近,進而使用不同高度的條件進行機翼釋放彈體數值模擬。機翼分別在1200公尺、6000公尺、11600公尺處釋放MK-82彈體,探討機翼分離彈體的軌跡,另外加上前彈射力四倍、前彈射力八倍分析其物理變化。當前彈射
力增大時,X軸向後移動量趨勢接近;Y軸向翼尖偏移量隨著前彈射力增加而稍微減少;Z軸向下掉落量則隨著前彈射力增加而明顯增加。
Applied Heath Care Analytics: Enabling Transformative Health Care Through Data Science, Machine Learning, and Cognitive Computin
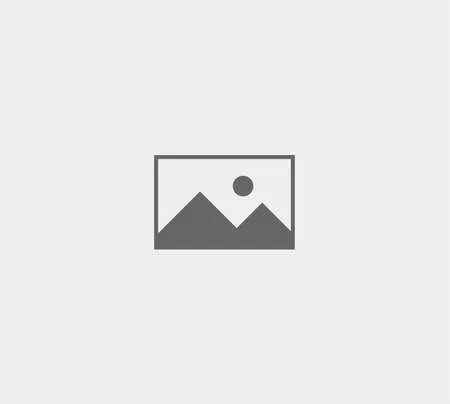
為了解決Machine learning sto 的問題,作者Albert, Mark/ Petrov, Plamen/ Ronanki, Rajeev 這樣論述:
The healthcare systems in the US and globally are undergoing a period of rapid transformation. Medical technology breakthroughs, economic pressures and demographic trends are driving that transformation, but key enablers and catalysts for those changes are advancements in Analytics, Data Science,
Cognitive Computing, and Machine Learning. Massive volumes of data are created during regular healthcare administration, delivery, and research operations; additionally, outside the medical community people produce data as part of their daily activities and social interactions that can be mined for
medical use. How can this data be put to use in an ethical way respecting privacy and security to achieve the goal of high quality, accessible and affordable Healthcare? Advanced analytics and cognitive computing are a big part of the answer. In Applied Heath Care Analytics, the authors provide a c
oncise yet comprehensive review of the key enabling tech and explain how those technologies are becoming the backbone of the Healthcare of tomorrow.
醫療影像辨識新興技術預測-以專利分析法探討
為了解決Machine learning sto 的問題,作者蔡志成 這樣論述:
本研究以智慧醫療中的影像辨識技術為主題,以專利分析法和技術生命週期探討智慧醫療影像辨識技術相關的9項技術趨勢發展。應用國際專利分類號(IPC)、關鍵字和通過檢核之公告專利做檢索,以國際專利分類號探討智慧醫療中影像辨識技術所重視之分類為何種影像辨識技術。本研究以智慧醫療影像辨識技術相關的9項技術累積之專利數,作為衡量技術績效之專利指標,以費雪成長模型(Fisher-Pry Growth Model)和羅吉斯成長模型(Logistic Gowth Model),描述技術生命週期和衡量技術參透比率。本研究使用以下專利技術與分類做檢索,且子技術又分為兩大類圖像數據分析(Image Data Anal
ysis)包含3D立體(Three-Dimensional)、終端(Terminal)、像素(Pixel)、監控器(Monitor),而另一類影像數據採集(Image Data Collection)包含醫學影像 (Medical image)、解剖(Anatomical)、超音波(Ultrasound)、圖像數據(Image data)、外科手術(Surgical)等,研究結果表示醫療影像辨識目前處於成長階段,眼科光學影像(Ophthalmic Optical Imaging)及圖像顯示器(Image Display)……等,是近年來發展技術的重點,加入了包含AI與非AI以及純AI相關醫療影
像辦識專利費雪成長模型比較,相較於非AI包含AI的新加入技術時間往後了大約10年發展時間,亦即未來還有很大的成長空間。
想知道Machine learning sto更多一定要看下面主題
Machine learning sto的網路口碑排行榜
-
-
#2.Stop explaining black box machine learning models for high ...
There has been an increasing trend in healthcare and criminal justice to leverage machine learning (ML) for high-stakes prediction applications ... 於 www.nature.com -
#3.Leveraging Machine Learning for Fraud Prevention | PayPal US
PayPal uses machine learning models and comprehensive data sets to help merchants detect and prevent fraud. Learn about our approach to ... 於 www.paypal.com -
#4.STOP Learning Machine Learning - YouTube
Focus on the SKILLS you need right now!!! If you're interested in the fastest path to a career in machine learning or any other data role my ... 於 www.youtube.com -
#5.Machine Learning Takes Safety to The Next Level
Construction technology expert Michael “MC” Contreras shares how we're training AI to make the jobsite even safer. 於 stobuildinggroup.com -
#6.When to Stop Training your Deep Learning Model
If the training is stopped before the optimal time, the model will not have had time to learn the most important features from the training set ... 於 towardsdatascience.com -
#7.Stop Experimenting With Machine Learning And Start Actually ...
Some 87% of corporate projects do not get past the experiment phase and never make it into production. There's no need to reinvent the wheel ... 於 www.forbes.com -
#8.Is it possible to stop machine learning training and continue it ...
In frameworks like PyTorch or Tensorflow it is pretty simple. You can save the weights of your model and then to restore those weights later ... 於 stackoverflow.com -
#9.The Mechanism of Prediction Head in Non-contrastive Self ...
Machine Learning Department ... non-contrastive learning: stop-gradient operation and output ... also incorporated the stop-gradient into their theory, ... 於 openreview.net -
#10.Removing stop words with NLTK in Python - GeeksforGeeks
Machine Learning Tutorial · Data Analysis Tutorial ... Stop Words: A stop word is a commonly used word (such as “the”, “a”, “an”, “in”) that ... 於 www.geeksforgeeks.org -
#11.Stop machine learning anomaly detection - Elastic
You must have manage_ml , or manage cluster privileges to stop datafeeds. For more information, see Security privileges. A datafeed can be started and stopped ... 於 www.elastic.co -
#12.How AI and Deep Learning stop Zero-Day cyber attacks
Learn how to use AI, machine learning, deep learning and automation to prevent the most sophisticated supply chain and zero-day cyber security threats. 於 pages.checkpoint.com -
#13.Utilization of machine learning algorithms to support retail ...
This machine-learning algorithm uses data from points where the phenomenon ... but is identified on the basis of the machine learning algorithms driven by ... 於 scholarworks.uni.edu -
#14.Adversarial attacks in machine learning: What they are and ...
Adversarial machine learning, a technique that attempts to fool models with ... into wrongly classifying a stop sign as a speed limit sign. 於 venturebeat.com -
#15.Deep Learning vs. Machine Learning – What's The Difference?
If the STOP sign image recognition was a more traditional machine learning algorithm, a software engineer would manually choose features and a ... 於 levity.ai -
#16.What are Stopwords in Machine Learning? | Aman Kharwal
Stop words are commonly used words that are excluded from searches to help index and crawl web pages faster. Some examples of stop words are: “a ... 於 thecleverprogrammer.com -
#17.Machine learning - Universitat de València
Machine learning, simulation, reinforcement learning, medical act/decision support systems. Manager UV. Martinez Gil, Francisco; PDI-Titular d'Universitat ... 於 www.uv.es -
#18.Einstein Send Time Optimization - Salesforce Help
Marketing Cloud Einstein Send Time Optimization (STO) determines the best time to send a message. Using machine learning, Einstein predicts optimal send t. 於 help.salesforce.com -
#19.Stop Using AI and ML Interchangeably - InformationWeek
The definitions of artificial intelligence and machine learning have evolved, so it's important for everyone to understand the distinctions. 於 www.informationweek.com -
#20.Stop Enrolling in Boring Courses! Teach Yourself Sci-Kit ...
Originally published on Towards AI. Discover an Engaging Way to Learn Sci-kit Learn: Master Machine Learning and Algorithms with the Power ... 於 towardsai.net -
#21.ML-based Mouse Tracking Enhances Adult ADHD Diagnosis ...
Machine Learning Stop Signal Test (ML-SST): ML-based Mouse Tracking Enhances Adult ADHD Diagnosis. 於 www.academia.edu -
#22.Log in - Facebook
Machine Learning http://goweb.com/ #machinelearning #sto #crypto #goweb. Галина Шуванева and 54 others. 55. . . Related Pages. Chelo Photo Art. 於 m.facebook.com -
#23.Solving the online batching problem using deep reinforcement ...
A Deep Reinforcement Learning approach to solve the order batching problem. ... An StO workstation can be used for picking-by-order and picking-by-batch and ... 於 www.sciencedirect.com -
#24.Early stopping - Wikipedia
In machine learning, early stopping is a form of regularization used to avoid overfitting when training a learner with an iterative method, such as gradient ... 於 en.wikipedia.org -
#25.Artificial Intelligence & Machine Learning Tutorials ... - Mindmajix
You are at the right place to learn and master AI and Machine Learning, a one-stop learning hub to help every individual ace AI and Machine Learning ... 於 mindmajix.com -
#26.Artificial Intelligence + Machine Learning: Stop Pretending
Artificial Intelligence will eliminate millions of jobs. It's inevitable. ... Artificial Intelligence + Machine Learning: Stop Pretending. 於 www.linkedin.com -
#27.ML- J-DP4: An Integrated Quantum Mechanics-Machine ...
ML- J-DP4: An Integrated Quantum Mechanics-Machine Learning ... at the cheapest HF/STO-3G level enhanced using machine learning (ML), ... 於 pubmed.ncbi.nlm.nih.gov -
#28.Can Machine Learning Stop the Coronavirus from Spreading?
This is because of the use of 'Artificial Intelligence' and its unique sub-field of 'Machine Learning'. However, to be able to stop something bad happening ... 於 aijourn.com -
#29.nickbild/go_motion: Simplify stop motion animation ... - GitHub
Simplify stop motion animation with machine learning. - GitHub - nickbild/go_motion: Simplify stop motion animation with machine learning. 於 github.com -
#30.Mastering Stop Motion Through Machine Learning | Hackaday
So while we can say its a quicker pace than with traditional stop motion production, it certainly isn't fast. Machine learning isn't the only ... 於 hackaday.com -
#31.Stop words removal | NLP | Bag of words - thatascience
Stop words are words like a, an, the, is, has, of, are etc. · Therefore removing stop words helps build cleaner dataset with better features for machine learning ... 於 thatascience.com -
#32.Stop and start a VM | Compute Engine Documentation
Essentially, a stopped VM resets to its power-on state and no data is saved. Stop a VM if you want to change the machine type, add or remove attached disks, ... 於 cloud.google.com -
#33.TrojanNet – a simple yet effective attack on machine learning ...
For instance, an attacker can fool the image processor of a self-driving car into bypassing a stop sign or mistaking it for a speed limit sign. 於 portswigger.net -
#34.Machine learning based prediction of metal hydrides for ...
The openly available database provided by the US Department of Energy on hydrides for hydrogen storage were analyzed through supervised machine learning to ... 於 www.researcher-app.com -
#35.Unsupervised Machine Learning in the Military Domain
Acquire new techniques for incremental learning in military applications. This activity will contribute to NATO's vision on Unsupervised Machine ... 於 www.sto.nato.int -
#36.Machine Learning Takes Safety to The Next Level - STOBG
Machine learning takes safety to the next level. Construction technology expert Michael “MC” Contreras shares how we're training AI to make ... 於 stobgtheword.com -
#37.What are Stop Words? - Kavita Ganesan, PhD
Supervised machine learning – removing stop words from the feature space · Clustering – removing stop words prior to generating clusters · Information retrieval – ... 於 kavita-ganesan.com -
#38.How to become a machine learning engineer - TikTok
#tech #techtok # machinelearning #datascience #techcareer #ai ... Become a Machine Learning Engineer | PhD Research: Machine Learning Social ... 於 www.tiktok.com -
#39.Overfitting in Machine Learning and Computer Vision
It indicates that the model is overfitting, and you can stop the training. Learning curve for an overfit model, Image Source. How to Prevent ... 於 blog.roboflow.com -
#40.MLGen: Generative Design Framework Based on Machine ...
MLGen integrates machine learning into the generative design practice. ... a short introduction of structural topology optimization (STO) is ... 於 www.mdpi.com -
#41.Send Time Optimization - Iterable Support Center
Send Time Optimization (STO) is an Iterable AI feature that helps send email and ... Iterable updates STO machine learning models once per week, using the ... 於 support.iterable.com -
#42.When Machine Learning Goes Off the Rails
Do the algorithm's errors affect minorities or other groups in particular? Can a continuous monitoring approach establish “guardrails” that stop the algorithm ... 於 hbr.org -
#43.Machine Learning: Or How I Learned To Stop Worrying and ...
What does the rapid rise of machine learning and AI mean for today's technology landscape? 於 www.profocustechnology.com -
#44.Manage a compute instance - Azure Machine Learning
Learn how to manage an Azure Machine Learning compute instance. ... In this article, you learn how to start, stop, restart, delete a compute ... 於 learn.microsoft.com -
#45.Real-time anomaly detection in computer networks using ...
Real-time detection of anomalies in computer networks with methods of machine learning: Stop the (data)-thief! · Some basics · Feature engineering ... 於 www.inovex.de -
#46.Machine Learning: New and Collected Sto..., Howey, Hugh
Author:Howey, Hugh. Machine Learning: New and Collected Stories. All of our paper waste is recycled within the UK and turned into corrugated cardboard. 於 www.ebay.com -
#47.Protecting smart machines from smart attacks
The Stop sign has been modified to change its meaning to systems relying ... “If machine learning is the software of the future, we're at a ... 於 www.princeton.edu -
#48.Tara ML?: A Zero-to-Hero Machine Learning Journey
Google Developer Student Clubs Polytechnic University Of The Philippines, Sto. Tomas, Batangas Branch presents Tara ML?: A Zero-to-Hero Machine Learning ... 於 gdsc.community.dev -
#49.Smart Train Operation Algorithms Based on Expert ...
This article proposes two smart train operation (STO) algorithms by integrating the expert knowledge with reinforcement learning algorithms. 於 ieeexplore.ieee.org -
#50.STO: “Machine Learning and Artificial Intelligence for Military ...
STO : Grupo de investigação exploratório sobre “Machine Learning and Artificial Intelligence for Military Vehicle Design”. 於 www.iddportugal.pt -
#51.G-STO: Sequential Main Shopping Intention Detection via ...
arXiv - CS - Machine Learning Pub Date : 2023-06-25 , DOI: arxiv- ... To fill this gap, we propose a graph-regularized stochastic Transformer method, G-STO. 於 www.x-mol.com -
#52.Support Vector Machine Active Learning ... - ACM Digital Library
Support Vector Machine Active Learning with Application sto Text Classification. Applied computing · Document management and text processing. 於 dl.acm.org -
#53.Send Time Optimization - Overview - Emarsys Help
Improved STO algorithm: How does it work? STO uses machine learning to analyze your contacts' behavior and identify the times when they are most ... 於 help.emarsys.com -
#54.Natural Language Processing with Optimal Deep Learning ...
Besides, STODL-FNDC model employs Deep Belief Network (DBN) approach for detection as well as classification of fake news. Finally, STO algorithm is ... 於 www.techscience.com -
#55.Roadmap on emerging hardware and technology for machine ...
State-of-the-art hardware for machine learning such as the central processing unit, ... Recently in situ surface potential profiles of STO/YSZ multilayer ... 於 iopscience.iop.org -
#56.Keras: Starting, stopping, and resuming training
Learning how to start, stop, and resume training a deep learning model is a super important skill to master — at some point in your deep ... 於 pyimagesearch.com -
#57.Machine learning in computational NMR-aided structural ...
These were coupled with NMR chemical shift predictions at the cheapest HF/STO-3G level, enhanced by machine learning (ML). 於 www.frontiersin.org -
#58.How to train your robot with deep reinforcement learning
Deep reinforcement learning (RL) has emerged as a promising approach for ... The human can reset the scene, stop the robot in unsafe ... 於 journals.sagepub.com -
#59.G-STO: Sequential main shopping intention ... - Amazon Science
https://www.amazon.science/publications/g-sto- ... Are you excited about understanding the state-of-the-art Machine Learning, Natural Language Processing, ... 於 www.amazon.science -
#60.Early Stopping to avoid overfitting in neural network- Keras
Early stopping is a method that allows you to specify an arbitrarily large number of training epochs and stop training once the model performance stops ... 於 medium.com -
#61.NLP – Stop Words - Study Machine Learning
Stop words are the words which are very common in text documents such as a, an, the, you, your, etc. The Stop Words highly appear in text documents. 於 studymachinelearning.com -
#62.Chapter 3 Stop words
Chapter 3 Stop words | Supervised Machine Learning for Text Analysis in R. 於 smltar.com -
#63.SPECIALIST PROGRAM IN STATISTICS (SCIENCE)
The Statistical Machine Learning and Data Science Stream focuses on applications of statistical ... STAC53H3 and STAD29H3, 1.0 credit must be STA courses. 於 utsc.calendar.utoronto.ca -
#64.STO Operating System - Mobideo
Can Digital Fundamentally Change STO Manageability? ... Machine learning algorithms make processes flexible and intelligent, transforming agility and ... 於 mobideo.com -
#65.hCaptcha - Stop more bots. Start protecting privacy.
Security First. Protect your services from scraping, account takeovers, credential stuffing, and spam with our advanced machine learning and humanity ... 於 www.hcaptcha.com -
#66.Udacity: Learn the Latest Tech Skills; Advance Your Career
Top Skill Collections · Data Engineering Courses · Neural Networks and Deep Learning Courses · Python Courses · SQL Courses · Product Management Courses · JavaScript ... 於 www.udacity.com -
#67.Machine learning for fraud detection - Ravelin
Old school fraud detection; Why is machine learning suited to fraud detection? ... Human insight is key to stop fraud attacks and limit the negative impact. 於 www.ravelin.com -
#68.How can machine learning stop email breaches | DLP - Egress
It's possible to prevent email breaches automatically using machine learning security solutions. Learn how in this guide. 於 www.egress.com -
#69.d2l-en.pdf - Dive into Deep Learning
10.7 Sequence-to-Sequence Learning for Machine Translation ... the returned value includes the first index (start) but not the last (stop). 於 d2l.ai -
#70.Machine Learning Gives Santa Cruz Traffic the Green Light
The Cloud Brigade team knew a Machine Learning solution would deliver a ... From a one stop-light town to a complex traffic light system ... 於 www.cloudbrigade.com -
#71.Machine Learning Archives - I Don't Know, Read The Manual
Stop words are the words that are filtered out when a computer is doing natural language processing. Which words are stop words? There is no single list of ... 於 www.idkrtm.com -
#72.Potential of AI and Machine Learning to Stop Bot Attacks
The vast majority know about automated bots like AI powered chatbots are really software applications that can utilize artificial ... 於 www.analyticsinsight.net -
#73.How 'Coraline' studio Laika uses Intel's AI to perfect stop ...
Laika is using a new Intel AI tool to clean up stop motion animation. ... Laika has partnered with Intel to use machine learning tools to ... 於 finance.yahoo.com -
#74.[PyTorch] Use Early Stopping To Stop Model Training At A ...
Early stopping is a technique applied to machine learning and deep learning, just as it means: early stopping. In the process of supervised ... 於 clay-atlas.com -
#75.Application of Machine Learning to Sporadic Experimental ...
Schematic workflow of modern data analytics for predicting hc. a) Experimental hc values of perovskite oxide thin films on STO, LAO, and LSAT (001) substrates ... 於 arxiv.org -
#76.Support Vector Machine Active Learning with ... - ResearchGate
Abstract. Support vector machines have met with significant success in numerous real-worldlearning tasks. However, like most machine learning algorithms, they ... 於 www.researchgate.net -
#77.Detecting Stop Signs and Traffic Signals - Lyft Engineering
I designed and productionized a deep-learning based algorithm that predicts where Traffic Control Elements (TCE), such as stop signs and ... 於 eng.lyft.com -
#78.Advancing Cyber Assurance with Deep Learning - DTIC
1 NATO STO, 'IST-183 Research Workshop on Deep Machine Learning For Cyber Defense (Virtual)', NATO Science & Technology. 於 apps.dtic.mil -
#79.My machine learning model does not learn. What should I do?
If you work with data in general, and machine learning algorithms in ... Every once in a while stop what you are doing and dedicate some ... 於 www.kdnuggets.com -
#80.Stoichiometric growth of SrTiO3 films via Bayesian ...
Perovskite insulator SrTiO3 (STO) is expected to be applied to the ... a new Bayesian optimization (BO)-based machine learning method that ... 於 pubs.aip.org -
#81.Machine Learning Stop Signal Test (ML-SST)
Machine Learning Stop Signal Test (ML-SST): ML-based Mouse Tracking Enhances Adult ADHD Diagnosis. 2019, pp. 1-5,. DOI Bookmark: 10.1109/ACIIW.2019.8925073. 於 www.computer.org -
#82.Machinelearning Stories - Wattpad
Unveiling How Machine Learning Sto...by Parangat Technologies. 801. In essence, machine learning operates as a vigilant guardian against spam, ... 於 www.wattpad.com -
#83.How Artificial Intelligence Learns Through Machine Learning ...
Artificial intelligence and machine learning algorithms are ever-present in the ... However, it does not stop learning at this point. 於 www.spiceworks.com -
#84.Most common method for deciding when to stop training a ...
So, the methods involving "summing" have been cut. machine-learning · neural-networks · gradient-descent · Share. 於 stats.stackexchange.com -
#85.What is early stopping? - Educative.io
Due to the advancements in machine learning, this method is pretty obsolete. 2. Stop when the loss function update becomes small 於 www.educative.io -
#86.Options for training deep learning neural network - MathWorks
An epoch is a full pass through the entire data set. During training, you can stop training and return the current state of the network by clicking the stop ... 於 www.mathworks.com -
#87.AI and Machine Learning: Stop Pretending - INDIAai
Home. map-forward. Videos. common-map-forward. AI and Machine Learning : Stop Pretending. HubSpot Marketing. 344K subscribers. 於 indiaai.gov.in -
#88.Use Early Stopping to Halt the Training of Neural Networks At ...
In this tutorial, you will discover the Keras API for adding early stopping to overfit deep learning neural network models. After completing ... 於 machinelearningmastery.com -
#89.Connections between robust statistical estimation, robust ...
... stochastic optimization, and robust machine learning problems ... The two-stage STO problems with quantile-based criteria can be ... 於 pure.iiasa.ac.at -
#90.Artificial Intelligence is a better approach to risk than stop-loss ...
The alliance combines Deloitte's business insights in cognitive technologies with Kira Systems' advances in machine learning in creating models that quickly " ... 於 www.pinterest.com -
#91.In London? See our work on Adversarial Machine Learning at ...
Visitors examine a display that shows a stop sign with stickers at the top and bottom. Research exploring adversarial machine learning, or the ... 於 techpolicylab.uw.edu -
#92.A Machine Learning Approach to Target Gain & Stop Loss
Reinforcement learning has appealing theoretical properties. An agent learns directly from data to choose exit thresholds that maximize a user-specified reward. 於 neuravest.net -
#93.Simplify stop motion animation with machine learning
Go Motion simplifies stop motion animation with machine learning. A CSI camera is connected to a Jetson Xavier NX. 於 forums.developer.nvidia.com -
#94.Machine learning analysis of perovskite oxides grown by ...
Details of the film growth conditions and MBE configuration for all samples are provided in the supplementary material. RHEED patterns for STO and LNO films ... 於 www.osti.gov -
#95.A Deep Reinforcement Learning Approach for Automated ...
In this work Deep Reinforcement Learning is applied to trade bitcoin. ... used in defining trading strategies: stop-loss and take-profit. 於 inria.hal.science