address的問題,我們搜遍了碩博士論文和台灣出版的書籍,推薦Puleo, Stephen寫的 Voyage of Mercy: America’’s Mission to Save the Irish 和Khor, Cheng Seong的 Model-Based Optimization for Petroleum Refinery Configuration Design都 可以從中找到所需的評價。
這兩本書分別來自 和所出版 。
國立陽明交通大學 資訊科學與工程研究所 謝秉均所指導 謝秉瑾的 貝氏最佳化的小樣本採集函數學習 (2021),提出 address關鍵因素是什麼,來自於貝氏最佳化、強化學習、少樣本學習、機器學習、超參數最佳化。
而第二篇論文國立陽明交通大學 資訊科學與工程研究所 陳冠文所指導 林正偉的 基於維持局部結構與特徵⼀致性之改善點雲語意分割方法 (2021),提出因為有 三維點雲、點雲處理、語意分割、電腦視覺、深度學習的重點而找出了 address的解答。
Voyage of Mercy: America’’s Mission to Save the Irish
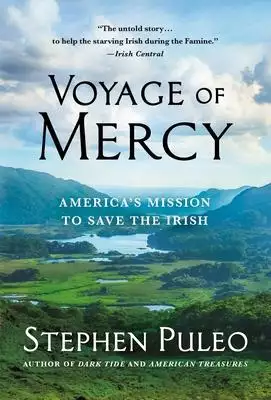
為了解決 address 的問題,作者Puleo, Stephen 這樣論述:
STEPHEN PULEO is a historian, teacher, public speaker, and the author of several books, including Dark Tide: The Great Boston Molasses Flood of 1919, American Treasures: The Secret Efforts to Save the Declaration of Independence, the Constitution, and the Gettysburg Address, and The Caning: The Assa
ult That Drove America to Civil War. A former award-winning newspaper reporter and contributor to American History magazine, the Boston Globe, and other publications, he holds a master’s degree in history and has taught at the University of Massachusetts-Boston and Suffolk University. He and his wif
e, Kate, reside in the Boston area.
address進入發燒排行的影片
貝氏最佳化的小樣本採集函數學習
為了解決 address 的問題,作者謝秉瑾 這樣論述:
貝氏最佳化 (Bayesian optimization, BO) 通常依賴於手工製作的採集函數 (acqui- sition function, AF) 來決定採集樣本點順序。然而已經廣泛觀察到,在不同類型的黑 盒函數 (black-box function) 下,在後悔 (regret) 方面表現最好的採集函數可能會有很 大差異。 設計一種能夠在各種黑盒函數中獲得最佳性能的採集函數仍然是一個挑戰。 本文目標在通過強化學習與少樣本學習來製作採集函數(few-shot acquisition function, FSAF)來應對這一挑戰。 具體來說,我們首先將採集函數的概念與 Q 函數 (Q
-function) 聯繫起來,並將深度 Q 網路 (DQN) 視為採集函數。 雖然將 DQN 和現有的小樣本 學習方法相結合是一個自然的想法,但我們發現這種直接組合由於嚴重的過度擬合(overfitting) 而表現不佳,這在 BO 中尤其重要,因為我們需要一個通用的採樣策略。 為了解決這個問題,我們提出了一個 DQN 的貝氏變體,它具有以下三個特徵: (i) 它 基於 Kullback-Leibler 正則化 (Kullback-Leibler regularization) 框架學習 Q 網絡的分佈(distribution) 作為採集函數這本質上提供了 BO 採樣所需的不確定性並減輕了
過度擬 合。 (ii) 對於貝氏 DQN 的先驗 (prior),我們使用由現有被廣泛使用的採集函數誘導 學習的演示策略 (demonstration policy),以獲得更好的訓練穩定性。 (iii) 在元 (meta) 級別,我們利用貝氏模型不可知元學習 (Bayesian model-agnostic meta-learning) 的元 損失 (meta loss) 作為 FSAF 的損失函數 (loss function)。 此外,通過適當設計 Q 網 路,FSAF 是通用的,因為它與輸入域的維度 (input dimension) 和基數 (cardinality) 無 關。通過廣
泛的實驗,我們驗證 FSAF 在各種合成和現實世界的測試函數上實現了與 最先進的基準相當或更好的表現。
Model-Based Optimization for Petroleum Refinery Configuration Design

為了解決 address 的問題,作者Khor, Cheng Seong 這樣論述:
An accessible, easy-to-read introduction to the methods of mixed-integer optimization, with practical applications, real-world operational data, and case studiesInterest in model-based approaches for optimizing the design of petroleum refineries has increased throughout the industry in recent yea
rs. Mathematical optimization based on mixed-integer programming has brought about the superstructure optimization method for synthesizing petroleum refinery configurations from multiple topological alternatives. Model-Based Optimization for Petroleum Refinery Configuration Design presents a detaile
d introduction to the use of mathematical optimization to solve both linear and nonlinear problems in the refining industry. The book opens with an overview of petroleum refining processes, basic concepts in mathematical programming, and applications of mathematical programming for refinery optimiza
tion. Subsequent chapters address superstructure representations of topological alternatives, mathematical formulation, solution strategies, and various modeling frameworks. Practical case studies demonstrate refinery configuration design, refinery retrofitting, and real-world issues and considerati
ons. Presents linear, nonlinear, and mixed-integer programming approaches applicable to both new and existing petroleum refineriesHighlights the benefits of model-based solutions to refinery configuration design problemsFeatures detailed case studies of the development and implementation of optimiza
tion modelsDiscusses economic considerations of heavy oil processing, including cash flow analysis of refinery costs and return on capitalIncludes numerical examples based on real-world operational data and various commercial technologiesModel-Based Optimization for Petroleum Refinery Configuration
Design is an invaluable resource for researchers, chemical engineers, process and energy engineers, other refining professionals, and advanced chemical engineering students.
基於維持局部結構與特徵⼀致性之改善點雲語意分割方法
為了解決 address 的問題,作者林正偉 這樣論述:
現今有許多研究探討如何運用深度學習方法處理三維點雲 (Point Cloud), 雖然有些研究成功轉換二維卷積網路到三維空間,或利用多層感知機 (MLP) 處理點雲,但在點雲語意分割 (semantic segmentation) 上仍無法到 達如同二維語意分割的效能。其中一個重要因素是三維資料多了空間維度, 且缺乏如二維研究擁有龐大的資料集,以致深度學習模型難以最佳化和容 易過擬合 (overfit)。為了解決這個問題,約束網路學習的方向是必要的。在 此篇論文中,我們專注於研究點雲語意分割,基於輸入點會和擁有相似局部 構造的相鄰點擁有相同的語意類別,提出一個藉由比較局部構造,約束相鄰 區域
特徵差異的損失函數,使模型學習局部結構和特徵之間的一致性。為了 定義局部構造的相似性,我們提出了兩種提取並比較局部構造的方法,以此 實作約束局部結構和特徵間一致性的損失函數。我們的方法在兩個不同的 室內、外資料集顯著提升基準架構 (baseline) 的效能,並在 S3DIS 中取得 目前最好的結果。我們也提供透過此篇論文方法訓練後的網路,在輸入點與 相鄰點特徵間差異的視覺化結果。