transformer的問題,我們搜遍了碩博士論文和台灣出版的書籍,推薦Doonan, Simon寫的 Transformer: How Lou Reed’s LGBTQ+ Love Songs Changed Us 和李金洪的 全格局使用PyTorch:深度學習和圖神經網路 實戰篇都 可以從中找到所需的評價。
這兩本書分別來自 和深智數位所出版 。
國立陽明交通大學 電機資訊國際學程 趙昌博所指導 黎文雄的 基於PPG信號和卷積神經網路測量血壓 (2021),提出 transformer關鍵因素是什麼,來自於光體積變化描記圖法 (PPG)、收縮壓 (SBP)、舒張壓 (DBP)、卷積神經網路 (CNN)。
而第二篇論文國立陽明交通大學 人工智慧技術與應用碩士學位學程 邱維辰所指導 葉鈺萱的 通過可微分深度渲染進行單眼360度佈局估算 (2021),提出因為有 360 影像應用、全景影像、佈局估計、場景理解、可微分深度渲染損失的重點而找出了 transformer的解答。
Transformer: How Lou Reed’s LGBTQ+ Love Songs Changed Us
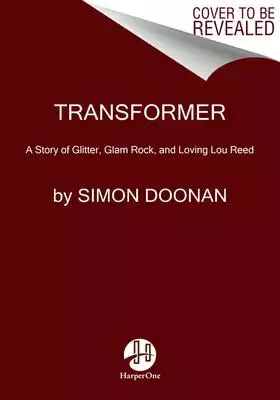
為了解決 transformer 的問題,作者Doonan, Simon 這樣論述:
In this funny and poignant memoir and cultural history, the television personality, columnist, and author of Drag pays homage to Lou Reed’s groundbreaking album Transformer on its fiftieth anniversary and recalls its influence on his coming of age and coming out through glam rock.In November 1972
, Lou Reed released his album, Transformer because he thought it was "dreary for gay people to have to listen to straight people’s love songs." That groundbreaking idea echoed with the times. That same year, Sweden was the first country to legalize gender-affirming surgery, and San Francisco struck
down employment discrimination based on sexual orientation.Sometimes an artistic creation perfectly aligns with a broader social and political history, and Transformer--with the songs "Walk on the Wild Side," "Perfect Day," and "Vicious"--perfectly captured its time. "Walk on the Wild Side" was bann
ed on radio across the country but became a massive hit when young people threatened to boycott stations that would not play it. The album’s cover featured a high-contrast image of Lou, flaunting a new mascara’d glamrock incarnation, shot by legend Mick Rock, thereby underscoring his intention to cr
eate a gay album.In Transformer, Doonan tells the story of how Lou Reed came to make the album with the help of David Bowie, and places its creation within the course of Reed’s life. Doonan offers first-hand testimony of the album’s impact on the LGBTQ+ community, recalling how it transformed his o
wn life as a 20-year-old working class kid from Reading, England, who had just discovered the joys of London Glam Rock and was sparked by the artistic freedom of Warhol’s The Factory. Transformer was a revelation--hearing Reed’s songs, Doonan understood how the world was changing for him and his fri
ends.A poignant, personal addition to modern music and LGBTQ+ history, Transformer captures a pivotal moment when those long silenced were finally given a voice. As transgender icon Candy Darling, highlighted in his lyrics, told Reed, "It’s so nice to hear ourselves."Transformer includes approximati
vely 16 pages of black-and-white and color photos.
transformer進入發燒排行的影片
基於PPG信號和卷積神經網路測量血壓
為了解決 transformer 的問題,作者黎文雄 這樣論述:
光體積變化描記圖法 (PPG) 是一種非侵入性和低成本的技術,現在被廣泛應用於許多血壓量測的研究中。儘管 PPG 信號的品質對血壓演算法的準確度有很大影響,但有關PPG信號的品質檢查並未得到重點關注。在實際量測時,除PPG信號外,反相PPG信號、雜訊、運動信號都會被採集,這些錯誤的信號如果無法去除就會導致錯誤的預測結果。因此,為了解決這個問題,本文提出了一種用於檢查PPG信號品質的新型卷積神經網路 (CNN) 模型,此使用新型CNN的品質檢查模型已被成功訓練和驗證,具有高精度和高性能。此外,本文還設計了另一個卷積神經網路模型來計算血壓,該模型可以自動檢測 PPG 信號中的重要特徵。最後,品質
檢查模型和 CNN 模型都成功嵌入到 Matlab 界面中,用於測量和收集更多數據,以便將來校準模型。
全格局使用PyTorch:深度學習和圖神經網路 實戰篇
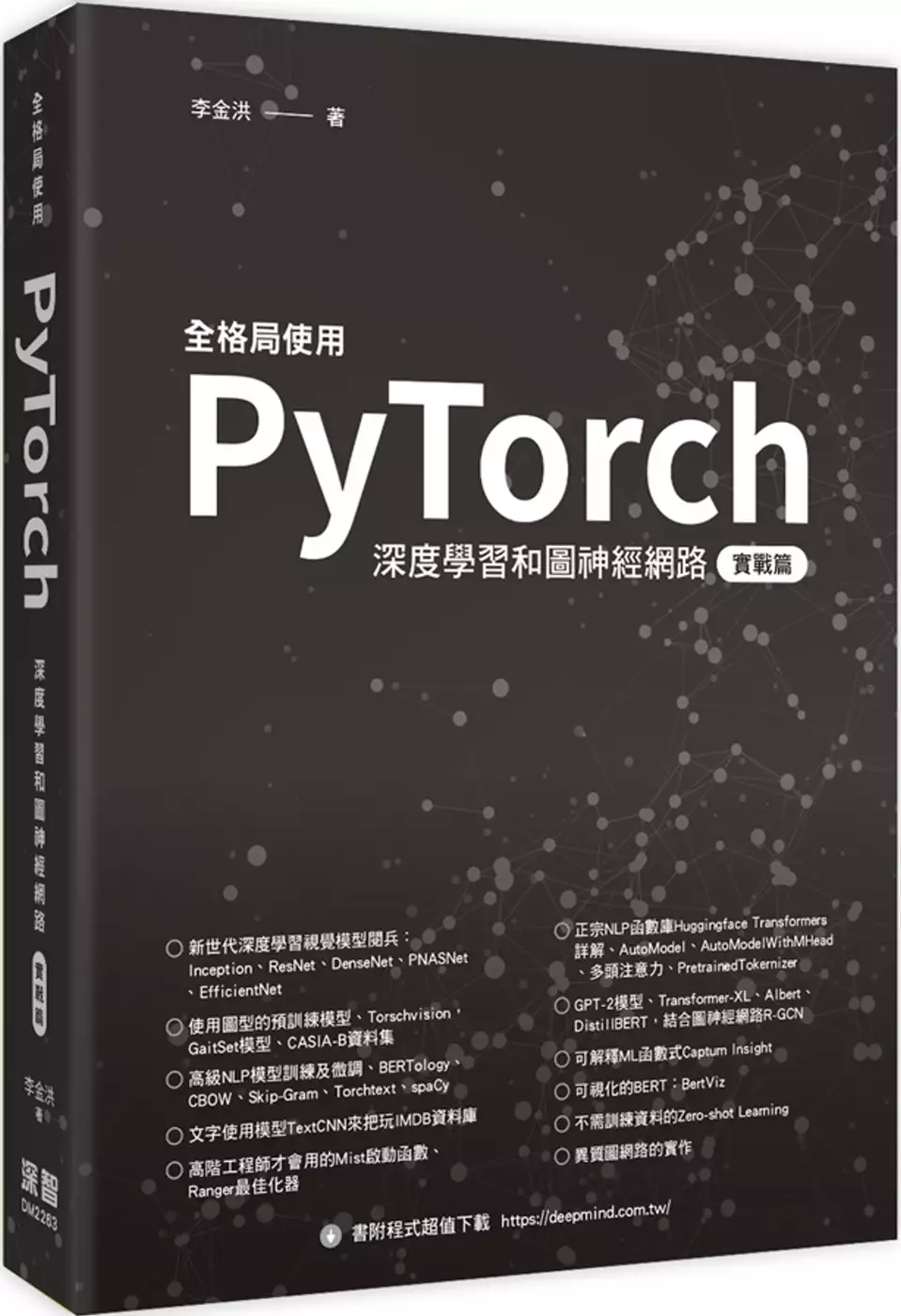
為了解決 transformer 的問題,作者李金洪 這樣論述:
熟悉基礎,精通實戰。 接續了上一本實戰篇的基礎,本書將介紹目前最流行的物體辨識和自然語言處理在圖神經網路上的最完整應用。當你熟悉了神經網路之後,接下來要精進的就是針對網路結果的強化及最佳化。在GNN的基礎上,針對目前最流行的視覺處理模型進行修改、架設及強化,並且實際應用在現有的平台上。本書的重點就是大量了使用現有的Python函數庫,並且應用了最新的資料集,讓你能真正看到資料套用在模型上的強大能力。在針對Pytorch的函數庫上,不但有視覺應用,更有號稱人工智慧明珠的NLP應用。使用了Torchtext以及NLP的唯一/最佳選擇Huggingface Transformers。而大家
耳熟能詳,但又不知道怎麼用的模型,包括GPT-2、Transformer-XL、ALBERT、ELECTRA、DistillBERT等,在書中都有詳細介紹。另外為了解開DL的神祕,本書也難得介紹了Captum套件,讓深度神經網路更具可解釋性。本書最後也不忘介紹ZSL、這種極少量資料就可訓練高精度模型的方法。有關異質圖神經網路部分,也有大量DGL和NetworkX的範例,實戰篇+基礎篇兩本書,要不充分了解GNN都不行。 本書特色 ~GNN 最強實戰參考書~ ●使用圖型的預訓練模型、Torschvision,GaitSet模型、CASIA-B資料集 ●高級NLP模型訓練及微調、BE
RTology、CBOW、Skip-Gram、Torchtext、spaCy ●文字使用模型TextCNN來把玩IMDB資料庫 ●高階工程師才會用的Mist啟動函數、Ranger最佳化器 ●正宗NLP函數庫Huggingface Transformers詳解、AutoModel、AutoModelWithMHead、多頭注意力、PretrainedTokernizer
通過可微分深度渲染進行單眼360度佈局估算
為了解決 transformer 的問題,作者葉鈺萱 這樣論述:
儘管在房間佈局估計任務上已經取得了顯著進展,大多數方法主要目標著重在減少 2D 像素坐標中的損失,而不是利用 3D 空間中的房間結構。為了重建 3D 房間的佈局,我們將 360 度佈局估計的任務公式化為預測全景圖地平線上深度的問題。具體來說,我們提出了可微分深度渲染 (Differentiable Depth Rendering) 的損失函數 (Loss Function),其過程可以使從佈局到深度預測的轉換可微分,從而使我們提出的模型可端到端地訓練,同時利用 3D 幾何信息,而無需提供真實深度。我們展示了我們的方法在眾多 360 佈局基準數據集上均達到了最先進的性能。此外,我們的公式可以對
深度數據集進行預訓練,從而進一步提高了我們的佈局估算模型的通用性。