Company irs的問題,我們搜遍了碩博士論文和台灣出版的書籍,推薦Copeland, Miles寫的 Two Steps Forward, One Step Back: My Life in the Music Business 和Siegel, Eric/ Davenport, Thomas H. (FRW)的 Predictive Analytics: The Power to Predict Who Will Click, Buy, Lie, or Die都 可以從中找到所需的評價。
另外網站medicaid-provider-distribution-instructions.pdf - HHS.gov也說明:companies for oral healthcare-related services, or (ii) owns (on the ... The applicant's Employer's Quarterly Federal Tax Return on IRS Form 941 for Q1 2020 ...
這兩本書分別來自 和所出版 。
佛光大學 管理學系 孫遜所指導 游朝欽的 中華郵政公司責任中心局經營績效評估之研究- 資料包絡分析法與平衡計分卡之運用 (2021),提出Company irs關鍵因素是什麼,來自於資料包絡分析法、平衡計分卡、績效評估、中華郵政、責任中心局。
而第二篇論文國立高雄大學 法學院博士班 廖義銘所指導 朱金藝的 有關數位平台反托拉斯規制問題之研究 (2021),提出因為有 數位平台、網路效應、多邊市場、獨占、結合、聯合、反托拉斯、限制競爭、經濟利益、消費者福利、競爭的重點而找出了 Company irs的解答。
最後網站Publication 542 (01/2022), Corporations - Internal Revenue ...則補充:If the corporation wishes to make this adjustment in some other way, it must get IRS approval. The corporation files a request for approval with its income ...
Two Steps Forward, One Step Back: My Life in the Music Business
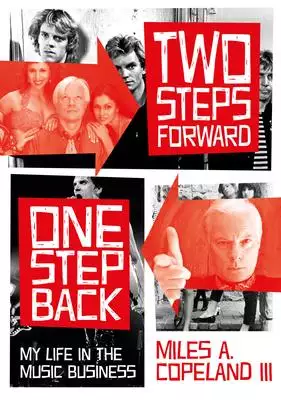
為了解決Company irs 的問題,作者Copeland, Miles 這樣論述:
Miles Copeland is a notorious icon and Svengali of the music business known primarily as manager for such superstars as The Police, Sting, Jools Holland, Squeeze, and The Bangles. He is also the founder of IRS Records, the most influential music label of the ’80s, which launched the careers of The G
o-Go’s, R.E.M., Fine Young Cannibals, The Alarm, Concrete Blonde, and Timbuk 3. As agent, manager, or record company, he has worked with artists as varied as the Sex Pistols, Keith Urban, Wishbone Ash, Zucchero, Buzzcocks, The Cramps, Wall Of Voodoo, Blondie, The Moody Blues, and a host of others.
中華郵政公司責任中心局經營績效評估之研究- 資料包絡分析法與平衡計分卡之運用
為了解決Company irs 的問題,作者游朝欽 這樣論述:
本研究旨在探討民國 105-109 年中華郵政股份有限公司 19 個責任中心局整體及個別經營績效、跨期生產力變動,並檢視環境變數對樣本責任中心局整體經營績效的影響。19 個責任中心局包含1家特等局、5家一等甲局、5家一等乙局、6家二等甲局及2家二等乙局。本研究整合資料包絡分析法與平衡計分卡,並採用產出導向確定區域模式評估樣本責任中心局整體經營績效與平衡計分卡四構面個別績效(學習與成長構面、內部流程構面、顧客構面與財務構面)。本研究續應用交叉效率模式找出最佳整體與個別績效之樣本責任中心局,再以麥氏生產力指數來分析樣本責任中心局跨期生產力變動及使用迴歸分析來檢視外在環境變數對樣本責任中心局整體經
營績效的影響。研究發現:(1) 樣本責任中心局有高內部流程績效,中高整體、學習與成長及顧客績效及低度財務績效;(2) 樣本責任中心局有 11 家連續 5 年處 IRS,有 6 家連續 5 年及 1 家連續 4 年處 DRS,有 1 家則是連續 5 年處於 CRS;(3) RC7為最佳整體與顧客績效責任中心局,RC1為最佳學習與成長及財務績效責任中心局,RC19為最佳內部流程績效責任中心局; (4) 高度整體經營績效責任中心局會有中高度的顧客績效,卻有低度學習與成長、內部流程及財務績效;(5) 樣本責任中心局平均跨期總要素生產力變動呈現成長,係因技術效率變動成長所致;(6) 樣本責任中心局所在地
屬性/城市其對整體績效具有正向且顯著的影響。
Predictive Analytics: The Power to Predict Who Will Click, Buy, Lie, or Die
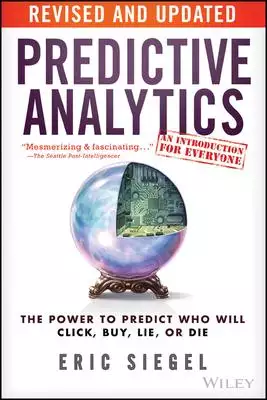
為了解決Company irs 的問題,作者Siegel, Eric/ Davenport, Thomas H. (FRW) 這樣論述:
"Mesmerizing & fascinating..." --The Seattle Post-Intelligencer"The Freakonomics of big data." --Stein Kretsinger, founding executive of Advertising.comAward-winning - Used by over 30 universities - Translated into 9 languagesAn introduction for everyone. In this rich, fascinating -- surprisi
ngly accessible -- introduction, leading expert Eric Siegel reveals how predictive analytics (aka machine learning) works, and how it affects everyone every day. Rather than a "how to" for hands-on techies, the book serves lay readers and experts alike by covering new case studies and the latest sta
te-of-the-art techniques.Prediction is booming. It reinvents industries and runs the world. Companies, governments, law enforcement, hospitals, and universities are seizing upon the power. These institutions predict whether you're going to click, buy, lie, or die. Why? For good reason: predicting hu
man behavior combats risk, boosts sales, fortifies healthcare, streamlines manufacturing, conquers spam, optimizes social networks, toughens crime fighting, and wins elections. How? Prediction is powered by the world's most potent, flourishing unnatural resource: data. Accumulated in large part as t
he by-product of routine tasks, data is the unsalted, flavorless residue deposited en masse as organizations churn away. Surprise This heap of refuse is a gold mine. Big data embodies an extraordinary wealth of experience from which to learn.Predictive analytics(aka machine learning) unleashes the
power of data. With this technology, the computer literally learns from data how to predict the future behavior of individuals. Perfect prediction is not possible, but putting odds on the future drives millions of decisions more effectively, determining whom to call, mail, investigate, incarcerate,
set up on a date, or medicate. In this lucid, captivating introduction -- now in its Revised and Updated edition -- former Columbia University professor and Predictive Analytics World founder Eric Siegel reveals the power and perils of prediction: What type of mortgage risk Chase Bank predicted bef
ore the recession.Predicting which people will drop out of school, cancel a subscription, or get divorced before they even know it themselves.Why early retirement predicts a shorter life expectancy and vegetarians miss fewer flights.Five reasons why organizations predict death -- including one healt
h insurance company.How U.S. Bank and Obama for America calculated the way to most strongly persuade each individual.Why the NSA wants all your data: machine learning supercomputers to fight terrorism.How IBM's Watson computer used predictive modeling to answer questions and beat the human champs on
TV's Jeopardy How companies ascertain untold, private truths -- how Target figures out you're pregnant and Hewlett-Packard deduces you're about to quit your job.How judges and parole boards rely on crime-predicting computers to decide how long convicts remain in prison.182 examples from Airbnb, the
BBC, Citibank, ConEd, Facebook, Ford, Google, the IRS, LinkedIn, Match.com, MTV, Netflix, PayPal, Pfizer, Spotify, Uber, UPS, Wikipedia, and more. How does predictive analytics work? This jam-packed book satisfies by demystifying the intriguing science under the hood. For future hands-on practition
ers pursuing a career in the field, it sets a strong foundation, delivers the prerequisite knowledge, and whets your appetite for more.A truly omnipresent science, predictive analytics constantly affects our daily lives. Whether you are a consumer of it -- or consumed by it -- get a handle on the po
wer of Predictive Analytics.
有關數位平台反托拉斯規制問題之研究
為了解決Company irs 的問題,作者朱金藝 這樣論述:
數位時代中,數位平台業者實施了牽涉到數位技術運用的一些新形態的限制競爭行爲,引起了對相關行爲反托拉斯規制方面的疑問與異見。藉由相關個案的累積,以美國、中國大陸晚近涉及數位經濟的案例作爲實務探討,研析數位經濟方面的反托拉斯法制議題。佐以蒐整相關主題的學理爭議,以限制競爭行爲三大態樣——獨占、結合與聯合行爲作爲區隔,探討數位平台業者所實施的競爭行爲於不同法律規制態樣中所生之法制適用問題與政策因應的重點議題與可能方向,對數位平台業者實施的限制競爭行爲之因應作出評斷。綜合來看,當前各地反托拉斯法制可以有效因應數位平台業者實施的限制競爭行爲,但鑑於此前對數位平台限制競爭行爲之規制多採放任自由主
義,面對數位經濟似乎已達到瓶頸時期、缺少創新動力,本文傾向於加強反托拉斯法之執行,主張在傳統以競爭效應爲主要特徵的反托拉斯適用上輔以消費者福利標準進行檢視,審慎選擇救濟措施,以防止將不利益轉嫁給消費者。 本文第一章對本研究背景、目的、方法等作初步介紹,第二章對數位平台分類與特徵等作簡要說明。第三章集中於立法目的之探討、美國反托拉斯法制沿革之介紹,明確後文對反托拉斯法制適用研究所採行的基本價值理念。第四章則討論數位領域供需規律與反托拉斯法制之基本原則。第五章主要對大陸以《反壟斷法》爲主的法律體系與台灣以《公平交易法》爲主的法律體系進行比較研究。第六章結合美國、大陸具市場力量的數位平台業者相
關案例進行剖析,對數位平台業者涉嫌濫用市場支配地位行爲之反托拉斯規制與法律政策調試進行研析;第七章則以同樣的模式研究數位平台業者結合。第八章則分析Uber平台及其勞務提供者的定性問題,探究是否可以運用反托拉斯法促使加強對勞務提供者權益的保障。第九章承接前章Uber案例分析的內容,研究數位平台以演算法爲工具的實施水平聯合行爲之反托拉斯規制。最後則爲本文結論章節,再次明確本文觀點以及總結對相關法制與政策發展的探討。
想知道Company irs更多一定要看下面主題
Company irs的網路口碑排行榜
-
#1.Michigan Department of Treasury - Taxes
Michigan Taxes, tax, income tax, business tax, sales tax, tax form, 1040, w9, treasury, withholding. 於 www.michigan.gov -
#2.Inland Revenue - Te Tari Taake
The final stage of Inland Revenue's multi-million-dollar programme to upgrade and simplify the tax system begins later this week. 21 June 2021. Business. 於 www.ird.govt.nz -
#3.medicaid-provider-distribution-instructions.pdf - HHS.gov
companies for oral healthcare-related services, or (ii) owns (on the ... The applicant's Employer's Quarterly Federal Tax Return on IRS Form 941 for Q1 2020 ... 於 www.hhs.gov -
#4.Publication 542 (01/2022), Corporations - Internal Revenue ...
If the corporation wishes to make this adjustment in some other way, it must get IRS approval. The corporation files a request for approval with its income ... 於 www.irs.gov -
#5.Income Taxes - The Balance Small Business
Learn all about filing business income taxes, including tax forms, ... How Do I Calculate Estimated Taxes for My Business? ... How to File IRS Form 4868. 於 www.thebalancesmb.com -
#6.Enterprise Statistics, 1963: Link of census establishment ...
Standard Procedure for Coding MultiIndustry Companies 25 b . Special Procedure for Coding Integrated Petroleum Extraction and Refining Companies 25 IRS ... 於 books.google.com.tw -
#7.Fixing the IRS: Paper Addiction Remains Agency's 'Kryptonite'
In part one of a series on the problems plaguing the IRS, ... The directive orders the agency to work with software companies to drum up a ... 於 news.bloombergtax.com -
#8.S Corporations | Internal Revenue Service
S corporations are responsible for tax on certain built-in gains and passive income at the entity level. To qualify for S corporation status, ... 於 www.irs.gov -
#9.3 Ways To Avoid Underpaying The IRS - Forbes
The IRS Underpayment of Estimated Tax penalty applies if you didn't ... W-4 to your company's payroll department—don't mail it to the IRS. 於 www.forbes.com -
#10.Foreign Companies IRS Tax Reporting for US Expats
Americans with foreign companies have to report them every year as part of their federal tax filing, including Americans living abroad. 於 brighttax.com -
#11.Department of the Secretary of State - Maine Corporations ...
IRS - Employee ID Numbers - An Employer Identification Number (EIN) is also known as a Federal Tax Identification Number. This link will provide information on ... 於 www.maine.gov -
#12.Tools | Internal Revenue Service
Track important business tax dates and deadlines right from your desktop. ... Register as an approved IRS business partner and conduct business ... 於 www.irs.gov -
#13.Minnesota Department of Revenue
Welcome to the Minnesota Department of Revenue website. We provide information to help you report and and pay Minnesota taxes, along with tax research, ... 於 www.revenue.state.mn.us -
#14.Entities | Internal Revenue Service
Unless a business meets the requirements listed below to be a qualified joint venture, a sole proprietorship must be solely owned by one ... 於 www.irs.gov -
#15.Businesses | Internal Revenue Service
As a small business or self-employed taxpayer, you should understand how the new tax law could affect your bottom line. Income (including Gains and Losses). 於 www.irs.gov -
#16.26 US Code § 162 - Trade or business expenses
rentals or other payments required to be made as a condition to the continued use or possession, for purposes of the trade or business, of property to which ... 於 www.law.cornell.edu -
#17.Link of Census Establishment and IRS Corporation Data
Standard Procedure for Coding Multi - Industry Companies b . Special Procedure for Coding Integrated Petroleum Extraction and Refining Companies IRS ... 於 books.google.com.tw -
#18.Australian Taxation Office: Home page
Check whether government COVID-19 grants, payments or stimulus you received is taxable or non-taxable. atotv ATO TV · Online services for business. 於 www.ato.gov.au -
#19.Swart Baumruk & Company, LLP
Swart Baumruk & Company is a full-service tax, accounting, payroll, and consulting firm serving Kissimmee and the Orlando area for more than 25 years. 於 www.sbc-cpa.com -
#20.Connecticut Department of Revenue Services - CT.gov
For Businesses. Starting a Business? File & Pay Online · e-Services Information · Sales & Use Tax · Corporation Business Tax · Business Entity Tax ... 於 portal.ct.gov -
#21.International Business | Internal Revenue Service
Review the various taxpayer identification numbers (TIN) the IRS uses to administer tax laws. Find the TIN you need and how to get it. Transfer ... 於 www.irs.gov -
#22.What Is the Internal Revenue Service (IRS)? - Investopedia
Most of the work of the IRS involves income taxes, both corporate and individual; it processed nearly 240 million tax returns in 2020. More than 94% of tax ... 於 www.investopedia.com -
#23.Small Business Tax Information | USAGov
Energy tax incentives for biodiesel and renewable diesel extended through 2022. Tax Relief in Disaster Situations. The Internal Revenue Service (IRS) offers ... 於 www.usa.gov -
#24.Small Business Forms and Publications - Internal Revenue ...
Download small business and self-employed forms and publications, or call 800-829-3676 to order forms and publications through the mail. 於 www.irs.gov -
#25.IRS Business Forms: A Comprehensive List - NerdWallet
IRS Form SS-4, also called the Application for Employer Identification Number, is not a business tax form per se. Unlike many of the other IRS business forms ... 於 www.nerdwallet.com -
#26.Welcome to revenue.ie
Buying and selling, Local Property Tax, Stamp Duty, Home Renovation Incentive, Help to Buy, rental income. Starting and running a business. Registering for tax, ... 於 www.revenue.ie -
#27.E-file and Payment Options - Department of Revenue
Service provider fees may apply. To pay an estimated payment or an extension payment for your Corporation Income Tax and/or Limited Liability Entity Tax (LLET):. 於 revenue.ky.gov -
#28.Tax Day Reminder: IRS Keeps Targeting the Poor - TriplePundit
As the IRS Keeps Targeting the Poor, Companies Gain Opportunities to Step Up and Assist Marginalized Citizens ... If you live in the U.S., your ... 於 www.triplepundit.com -
#29.Business Taxes | Internal Revenue Service
All businesses except partnerships must file an annual income tax return. Partnerships file an information return. The form you use depends on ... 於 www.irs.gov -
#30.Free File | Benefits.gov
Available exclusively at IRS.gov, IRS Free File makes doing your federal taxes less taxing, using guided tax preparation or Free File Fillable Forms that do ... 於 www.benefits.gov -
#31.Employer ID Numbers | Internal Revenue Service
An Employer Identification Number (EIN) is also known as a Federal Tax Identification Number, and is used to identify a business entity. 於 www.irs.gov -
#32.On Tax Day, Treasury Makes a Plea For More IRS Funding
The Internal Revenue Service started this tax season buried in a ... “The I.R.S. knew walking into this filing season that it did not have ... 於 www.nytimes.com -
#33.Forming a Corporation | Internal Revenue Service
A corporation generally takes the same deductions as a sole proprietorship to figure its taxable income. A corporation can also take special ... 於 www.irs.gov -
#34.GuideStar nonprofit reports and Forms 990 for donors ...
Easily search 1.8 million IRS-recognized tax-exempt organizations, and thousands of faith-based nonprofits · Gather insights on financials, people/leadership, ... 於 www.guidestar.org -
#35.SEC.gov | HOME
Small Business. Check out our new Small Biz site, with info to help companies that want to raise capital. 於 www.sec.gov -
#36.General Information & Available Resources - Florida ...
IRS – Business Tax Compliancy. The Internal Revenue Service is the federal government agency responsible for tax collection and tax law in the United States ... 於 dos.myflorida.com -
#37.有限責任公司
請參閱8832表【一般說明】的更多資訊。 Related Topics. Single Member Limited Liability Companies · LLC Filing as a Corporation or Partnership. 於 www.irs.gov -
#38.DOR Wisconsin Department of Revenue Portal
Whether you want to start a new business or expand a current one, BDGR can help you find resources to start, grow & thrive. 於 www.revenue.wi.gov -
#39.Lesson 1- Federal taxes and your new business - IRS Video ...
[music playing] Let's start first with the Federal Employer Identification Number or EIN. An EIN identifies tax returns filed with the IRS and, as a business ... 於 www.irsvideos.gov -
#40.IRS Principle Business Codes: A Guide for Managers - Indeed
Understand what an IRS principal business code is, why your business will need one and how it affects your business taxes. 於 www.indeed.com -
#41.SARS Home | South African Revenue Service
Small Business Information. Picture of shipping containers Customs and. Excise Updates. Picture of a briefcase full of documents Tenders. 於 www.sars.gov.za -
#42.Home Comptroller.Texas.Gov
This office strives to provide you the best possible services and resources to do business in Texas. This website provides you with easy access to tax forms ... 於 comptroller.texas.gov -
#43.IRS Careers |
A description of the business units can be found at: https://www.jobs.irs.gov/about/who/business-... ApplyShare. 於 www.jobs.irs.gov -
#44.Employment Development Department | California
Featured Links. Essential Needs · Help Fight Fraud · Public Records Request · Disaster-Related Services · California Business Portal · Quick Statistics ... 於 edd.ca.gov -
#45.Tax Day 2022: IRS website experiences major slowdown
The Internal Revenue Service's website experienced problems on Monday ... The IRS told FOX Business that its site was never down on Tax Day. 於 www.foxbusiness.com -
#46.Welcome to EFTPS online
... can pay any tax due to the Internal Revenue Service (IRS) using this system. ... U.S. Mail in five to seven business days at your IRS address of record. 於 www.eftps.gov -
#47.Department of Taxation and Finance
Log in to your Business Online Services account to Web File your sales tax returns. With Web File, you can schedule payments in advance, save your bank ... 於 www.tax.ny.gov -
#48.Tax Information For Corporations - Internal Revenue Service
This site provides e-file information for corporations that prepare and transmit their own electronic corporate income tax returns and those ... 於 www.irs.gov -
#49.Welcome to the Illinois Department of Revenue
Tax Types. Taxes, Excise tax, Value added tax, Property tax, Income tax, Corporate ... 於 www2.illinois.gov -
#50.Closing a Business | Internal Revenue Service
The IRS has resources that can help you navigate this. On this page, you'll find the steps you'll need to take to close your business from a ... 於 www.irs.gov -
#51.Welcome to GuamTax.com | The Official Web Site of the Guam ...
E-File 1096/1099; Renew Business License; Vehicle Safety Inspection Companies: submit Vehicle Inspection Reports. Vehicle Insurance Companies & Agents: submit ... 於 www.guamtax.com -
#52.Businesses | Internal Revenue Service
Corporations that elect to pass corporate income, losses, deductions, and credits through to their shareholders for federal tax purposes. 於 www.irs.gov -
#53.Internal Revenue Service - Wikipedia
This is partially due to the nature of the individual income tax category, containing taxes collected from working class, small business, self-employed, and ... 於 en.wikipedia.org -
#54.Virginia Tax: Home
Forms & Instructions · Individual Income Tax Filing · Estates, Trusts, and the Deceased · Sales and Use Tax · Employer Withholding · Corporation Income Tax ... 於 www.tax.virginia.gov -
#55.Pay1040.com - IRS Authorized Payment Provider
The trusted and secure way to make business tax return payments to the IRS with your credit or debit card for a low fee. 於 www.pay1040.com -
#56.TurboTax® Official Site: File Taxes Online, Tax Filing Made Easy
an audit. Every personal TurboTax return is backed by our Audit Support Guarantee for free guidance from a trained tax professional. IRS E-File ... 於 turbotax.intuit.com -
#57.NCDOR: Home Page
The NCDOR is committed to helping taxpayers comply with tax laws in order to fund public services benefiting the people of North Carolina. 於 www.ncdor.gov -
#58.FBR| Federal Board of Revenue - Government of Pakistan
Pakistan Doing Business Reforms ... Constitution of Committee for Resolution of Issues of Pharmaceutical Companies · Quota for Import of Raw Materials for ... 於 www.fbr.gov.pk -
#59.HM Revenue & Customs - GOV.UK
HMRC Charter. 4 November 2021 — Corporate report. This Charter explains what you can expect from us and what we expect from ... 於 www.gov.uk -
#60.IRS audits declined as businesses racked up tax breaks - The ...
Federal audits of corporate tax returns have plunged in recent years, letting big companies claim elaborate tax breaks with less government ... 於 www.washingtonpost.com -
#61.Internal Revenue Service | LinkedIn
Website: http://www.irs.gov. Industries: Government Administration. Company size: 10,001+ employees. Headquarters: Washington, District of Columbia. 於 www.linkedin.com -
#62.BIR Home - Bureau of Internal Revenue
See Tax Deadlines, Know How to Get a TIN, Know How to Register a Business, Know How to File Tax Return/Pay Taxes, Use BIR eServices, Access BIR Forms ... 於 www.bir.gov.ph -
#63.Large Business and International Tax Center - Internal ...
The Treasury Department and IRS are implementing Schedule M-3, “Net Income (Loss) Reconciliation for Corporations With Total Assets of $10 Million or More”. 於 www.irs.gov -
#64.Assistance for Small Businesses - Treasury
Small Business Tax Credit Programs · Emergency Capital Investment Program · Paycheck Protection Program · Bureaus · Inspector General Sites · U.S. Government Shared. 於 home.treasury.gov -
#65.Internal Revenue Service - Crunchbase Company Profile ...
Company Type Non-profit. Phone Number 1-202-283-1710. Internal Revenue Service is a government organization that provides tax administration services for ... 於 www.crunchbase.com -
#66.Treasury makes plea to Congress for more IRS funding
Business. Treasury makes plea to Congress for more IRS funding ... The Biden administration's proposals to provide the IRS with $80 billion ... 於 www.businessreport.com -
#67.Telephone Assistance Contacts for Business Customers
Business and Specialty Tax Line and EIN Assignment (800-829-4933) Regular Hours of Operation · Services: · Electronic Products and Services ... 於 www.irs.gov -
#68.About Form 7004, Application for Automatic Extension of Time ...
Information about Form 7004, Application for Automatic Extension of Time To File Certain Business Income Tax, Information, and Other Returns, ... 於 www.irs.gov -
#69.企業結構 - Internal Revenue Service
您的企業類型決定了您申報所得稅時必須使用的稅表。最常見的企業類型是獨資經營(Sole Proprietorship)、合夥公司(Partnership)、股份公司(Corporation)和 ... 於 www.irs.gov -
#70.Starting a Business | Internal Revenue Service
Find federal tax information for people starting a business, and information to assist in making basic business decisions. 於 www.irs.gov -
#71.IRAS
Inland Revenue Authority of Singapore (IRAS) is the Government agency responsible for the administration of taxes and enterprise disbursement schemes. 於 www.iras.gov.sg -
#72.Ogden Business Owner Sentenced to a Year in Prison for Tax ...
Fry further failed to pay to the IRS the employer portion of the trust fund taxes owed by his businesses. In total, from January 2013 to ... 於 www.justice.gov -
#73.Pay Taxes Electronically - DOR - IN.gov
INTIME provides access to manage and pay individual income, and various corporate and business tax obligations. For more information, visit INTIME. Ready to ... 於 www.in.gov -
#74.Our Business Divisions | IRS Careers
Our Business Divisions Your individual contributions can gain new purpose for your nation in many different ways at the IRS. In addition to a wide range of ... 於 www.jobs.irs.gov -
#75.How to Handle an IRS Business Tax Audit | H&R Block
IRS agents conduct most business tax audits in person. Business audits are usually comprehensive, covering income tax issues and other issues, such as ... 於 www.hrblock.com -
#76.Small Businesses Self-Employed | Internal Revenue Service
Small Business and Self-Employed Tax Center · Employer ID Numbers (EINs) · Forms and Publications · Self-Employment Taxes · E-file Employment Taxes ... 於 www.irs.gov -
#77.Filing and Paying Your Business Taxes - Internal Revenue ...
An Employer Identification Number (EIN), also known as a federal tax identification number, is used to identify tax reports to the IRS. Business ... 於 www.irs.gov -
#78.IRS tax day 2022: What happens if I file late or not at all? - Fast ...
The IRS knows this whole business of stimulus checks and rebate credits is far more complicated than it should be, so it recently updated its ... 於 www.fastcompany.com -
#79.Treasury Blames Audit Imbalance on Underfunding of IRS
Larsen Weighs Merging Tech Arms Into a $22 Billion Firm, Sources Say · A Merger Of L&T Infotech, Mindtree Is On The Cards · Baiju KaleshAnto Antony. 於 www.bloombergquint.com -
#80.IRS Revenue Ruling 59-60
The appraiser must exercise his judgment as to the degree of risk attaching to the business of the corporation which issued the stock, but that judgment must be ... 於 www.pvfllc.com -
#81.Tax Day 2022: IRS website slows down, experiences delays
Unfortunately, the IRS website slowed down, and several unhappy ... (Catch all the Business News, Breaking News Events and Latest News ... 於 economictimes.indiatimes.com -
#82.Forms for Corporations | Internal Revenue Service
More In File ... Report and pay FUTA tax if the corporation either: ... If you are an employer, you must file a quarterly Form 941 to report:. 於 www.irs.gov -
#83.Free Taxes: 8 Ways to File Your Tax Return for Free - CNET
IRS Free File and Free File Fillable Forms. The IRS partners with tax companies to provide its Free File Program, which offers free tax software ... 於 www.cnet.com -
#84.Louisiana Department of Revenue: Home Page
... which provides incentives for companies to hire unemployed young people from ... Make sure the Louisiana Department of Revenue and/or the IRS have not ... 於 revenue.louisiana.gov -
#85.Liquidators try to prevent Brockman-linked company's assets ...
An exempted Bermuda company linked to a Texan billionaire accused of a ... to prevent Brockman-linked company's assets being seized by IRS. 於 www.royalgazette.com -
#86.IRS | Facebook
IRS. 444961 likes · 4283 talking about this. Tax information and news from the Internal Revenue Service (IRS). If you're looking for the officia. 於 www.facebook.com -
#87.Employer W-2 Filing Instructions & Information - Social Security
... Semi-Annual Meeting between SSA, the Wage Reporting Community, and the IRS. ... Provide your name, company name, and email address for each attendee. 於 www.ssa.gov -
#88.An official website of the United States government - Internal ...
支付您的稅款。 獲取您的退稅狀態。 查找表格、說明和稅務問題的答案。 國稅局幫助您瞭解並履行您的聯邦稅務責任。 於 www.irs.gov -
#89.IRS Summary Collection Policy Impact on Small Business: ...
Situation 1 : In the fall of 1978 , a business associate of mine and I ... of the vendors and the Internal Revenue Service which would allow the company to ... 於 books.google.com.tw -
#90.International Rescue Committee (IRC)
The International Rescue Committee (IRC) responds to the world's worst humanitarian crises and helps people to survive and rebuild their lives. 於 www.rescue.org -
#91.IRS Levy | Business Tax Problems | 20/20 Tax Resolution
When it comes to your business facing a federal (IRS) levy, it's important to learn what a levy is, why it happens and what to do about it. 於 2020taxresolution.com -
#92.TIN Description US taxpayer identification numbers i - OECD
An EIN is issued by the IRS to sole proprietors, partnerships, ... Taxpayers with specific individual or business account questions should contact the ... 於 www.oecd.org -
#93.SOI Tax Stats - Corporation Tax Statistics - Internal Revenue ...
Publications, Other IRS Data and Related Links ; Browse for articles and tables from the following publications: For data on tax administration and other taxes, ... 於 www.irs.gov -
#94.Tax Relief Companies | Consumer Information - Federal Trade ...
IRS Help for Taxpayers. If you owe taxes, but can't pay the IRS in full, consider submitting an Installment Agreement Request (Form 9465) with your return ... 於 consumer.ftc.gov -
#95.A-Z Index for Business | Internal Revenue Service
Search for business topics like employer tax identification number (EIN) information, with the A-Z Index. Search by business type or subject. 於 www.irs.gov -
#96.Canada Revenue Agency
Personal, business, corporation, and trust income tax ... for your personal income taxes, business taxes, or some government programs ... 於 www.canada.ca -
#97.Register your business - SBA
To create an S corp, you'll need to file form 2553 with the IRS. Business registration documents sent to local, state, and federal agencies. Register with ... 於 www.sba.gov -
#98.IRS Investigating American Express Sales Pitches - WSJ
The pitch was part of a strategy to persuade business owners to sign up for costly payment services. AMEX IN FOCUS. Articles about the company's ... 於 www.wsj.com -
#99.State of NJ - Department of the Treasury - Division of Taxation
Make sure your new business is checking all the boxes, including permitting ... The IRS is sending out an important notice in January to those who received ... 於 www.state.nj.us