Party 2的問題,我們搜遍了碩博士論文和台灣出版的書籍,推薦Futsukaichi, Tofuro寫的 It’’s a Little Hard to Be a Villainess of an Otome Game in Modern Society (Light Novel) Vol. 2 和L’Amour, Louis的 Westward the Tide (Louis l’’Amour’’s Lost Treasures)都 可以從中找到所需的評價。
另外網站英語你我他-生活篇07 接人參加party(2) - 教育廣播電台 ...也說明:第7集英語你我他-生活篇07 接人參加party(2). 1. 131. 分享. 英語. 邀訪來賓:播出日期:2005/09/13. 接人參加party(2). 心得筆記. 播放時間00:00. 心得筆記內容.
這兩本書分別來自 和所出版 。
世新大學 資訊管理學研究所(含碩專班) 高瑞鴻所指導 陳慧姍的 系統移轉關鍵因素之實務研究 (2022),提出Party 2關鍵因素是什麼,來自於系統移轉程序、專案管理、關鍵成功因素。
而第二篇論文國立中正大學 資訊管理系研究所 胡雅涵、李珮如所指導 宋昇峯的 以監督式機器學習探討電子病歷中非結構化資料對早期預測中風後功能復原後果之價值 (2021),提出因為有 急性缺血性中風、電子病歷、功能復原後果、機器學習、敘述式臨床紀錄、自然語言處理、風險模型、預測的重點而找出了 Party 2的解答。
最後網站House Party 2 - 中文百科知識則補充:《House Party 2》是由George Jackson 、 Doug McHenry 執導的一部喜劇類電影。基本信息Estudantes Devem Estar Loucos, Os.....Portugal Kid'n Play - Det vilda ...
It’’s a Little Hard to Be a Villainess of an Otome Game in Modern Society (Light Novel) Vol. 2
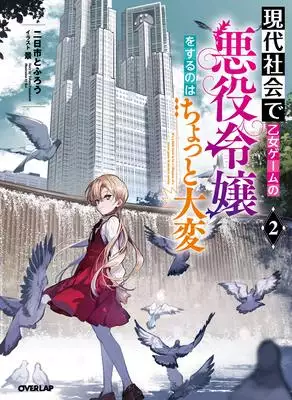
為了解決Party 2 的問題,作者Futsukaichi, Tofuro 這樣論述:
FOR THE SAKE OF THE FUTURE, I’LL DESTROY THE RIKKEN SEIYUU PARTY!After narrowly pulling the Keika Group through what appeared to be an imminent collapse, Runa has ascended to new heights as The Little Queen. Leveraging currency, cunning, and financial power, she continues to establish the Fuchiga
mi administration. However, danger and distrust lurk around every corner, leaving Runa to face still looming tragedy with her futuristic knowledge once again. And as this version of Japan looks towards the 21st century, Runa finds less and less time to save Japan from utter economic collapse. It see
ms that no matter what, the fate of Japan is set to occur once again.
Party 2進入發燒排行的影片
木曜日をお知らせします。
プリコネラジオ:https://hibiki-radio.jp/description/priconne_re/detail
チャンネル登録よろしくお願いします!→http://u0u0.net/KmNR
えるるぅと申します!
毎週木曜日プリコネラジオの日。
いつもご視聴いただきありがとうございます!
本日はハロウィン関連のアプデがたくさんありますので、イベント攻略とか頑張りましょう。
前回→https://youtu.be/9IhO-02b5Oc
Hi, I'm Eruru. I'm making "game play" videos. I love [Princess Connect Re:Dive] [THE IDOLM@STER CINDERELLA GIRLS] [BanG Dream! Girls Band Party!] [Granblue Fantasy]. I'm looking forward to seeing you in my videos or Live broadcast.
【基本的なこと】
初心者向け講座Part1から→https://youtu.be/D-vXmcrHtgE
クランバトル基礎知識→https://youtu.be/8Gwapnve0-c →https://youtu.be/iYJzy6Lx8oY
見たら始めたくなる動画→https://youtu.be/aYb1gQcN_0A
【キャラ育成関連】
キャラ育成優先度→https://youtu.be/4asOXdYY_wo
→https://youtu.be/1_Yn-YUlDLUHARD
回るべき場所解説→https://youtu.be/IF25xESGsvw
→https://youtu.be/Dmont82nFYg
各種コインの交換優先度→https://youtu.be/xe7sLWmIJqw
女神の秘石の賢い使い方→https://youtu.be/mco9nen_90E
専用装備について→https://youtu.be/bqcq6wUpRKg
→https://youtu.be/ZUHy1NyalO0
タンクとヒーラーについて→https://youtu.be/9-f0e1N3zIk
キャラの星上げについて→https://youtu.be/bzlc-XetBAc
【攻略関連】
ダンジョンEXⅡ解説→https://youtu.be/E6rsuwqIUKU
効率的なイベント攻略→https://youtu.be/E5jpIKouJbQ
イベントHARDボスワンパンの考え方→https://youtu.be/W-0Y9hzYCug
ルナの塔解説→https://youtu.be/5zldcsipwN8
ドロップ3倍・イベント・聖跡どれやる?→https://youtu.be/Nvazz4Vprws
【知っておくと良いこと等】
絶対ぶち当たる疑問ランキングTOP5→https://youtu.be/0z0jGl29A9Y
知っておきたい知識・小技5選→https://youtu.be/xfOp9Sd2Ca4
後悔しない装備集め講座→https://youtu.be/zSDH4J7NNqM
用語・略語解説→https://youtu.be/zQ1KHvJDVI0
マナの稼ぎ方について→https://youtu.be/zQX8b9j4s7Q
プリコネR動画リスト:https://youtu.be/3VOmg3PGj9g?list=PL_ygR7-1_wn1EaMt2RBokzLJVPE2SHNqv
プリンセスコネクト!Re:Dive公式サイト:https://priconne-redive.jp/
★ご連絡
eruru.urure☆gmail.com
※☆→@にしてください
★ Twitter
https://twitter.com/_eruru_
★Mildom
https://www.mildom.com/profile/10394665
ミルダムでも生放送やってます!
よければフォローよろしくお願いします。
★Twitch
https://www.twitch.tv/eruru
#プリコネR #PrincessConnect #アイテム交換キャラ
系統移轉關鍵因素之實務研究
為了解決Party 2 的問題,作者陳慧姍 這樣論述:
隨著時代變遷與新業務的多樣化需求,一個行之己久的系統,除了穩定運作之外,系統快速變更的能力也是必需的,面對業務需求的增加及新技術發展,系統移轉開發升級是必然面對的課題,系統移轉前有足夠的溝通及明確的專案範圍定義,可讓系統移轉開發達到事半功倍的效果。本研究目的在針對系統移轉實務開發過程、相關文獻及專案管理知識指南 (PMBOK Guide, A Guide to the Project Management Body of Knowledge),進行探討系統移轉關鍵指標因素及程式開發者規劃及應對,研究係採用專家訪談問卷並佐層級分析法研究方法分析推論其結果,達成研究結論,共計發出九份專家訪談問卷
,並回收九份,研究歸納結果顯示「系統移轉」關鍵要素計算出的權重比例前三項分別為「組織政策」、「專案目標」及「系統分析」。
Westward the Tide (Louis l’’Amour’’s Lost Treasures)
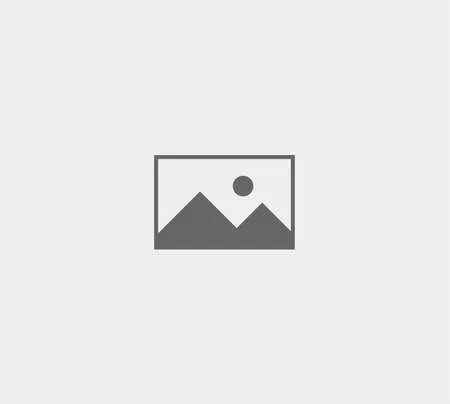
為了解決Party 2 的問題,作者L’Amour, Louis 這樣論述:
Louis L’Amour’s Lost Treasures is a project created to release some of the author’s more unconventional manuscripts from the family archives.Matt Bardoul was a good man to have as a friend and a bad one to make trouble with. He was also a single-minded drifter--until he met his match in an outspo
ken beauty named Jacquine Coyle. She was headed into the Bighorn Mountains with her father and an expedition in search of gold. After Matt signs on to join them, he discovers that there is a group of outlaws in the party--gunfighters and thieves that Matt wouldn’t trust for a minute. At first it’s u
nclear what they are planning, but before long Matt realizes that he’s the only man standing between innocent people and a brutal conspiracy of greed, lust, and cold-blooded murder. In Louis L’Amour’s Lost Treasures: Volumes 1 and 2, Beau L’Amour takes the reader on a guided tour through many of th
e finished and unfinished short stories, novels, and treatments that his father was never able to publish during his lifetime. L’Amour’s never-before-seen first novel, No Traveller Returns, faithfully completed for this program, is a voyage into danger and violence on the high seas.Additionally, ma
ny beloved classics are being rereleased with an exclusive Lost Treasures postscript featuring previously unpublished material, including outlines, plot notes, and alternate drafts. These postscripts tell the story behind the stories that millions of readers have come to know and cherish.
以監督式機器學習探討電子病歷中非結構化資料對早期預測中風後功能復原後果之價值
為了解決Party 2 的問題,作者宋昇峯 這樣論述:
中風是導致成人殘障的重要原因,中風功能復原後果的精準預測,能協助病人及家屬及早準備後續照顧事宜,衛生政策制定者也能依此預測結果適切規劃人力與資源,以投入中風病人的急性後期與中長期照護。目前的中風功能復原後果預測模型皆是以結構化資料建立,甚至最新使用數據驅動方式發展的機器學習預測模型依然是以結構化資料為主。相對的,照顧病人所製作的大量敘述式病歷文字紀錄,即非結構化資料,反而甚少被使用。因此,本研究的目的,即是使用監督式機器學習來探討非結構化臨床文字紀錄於急性缺血性中風後之初期預測功能復原後果之應用價值。在6176位2007年10月至2019年12月間因急性缺血性中風住院之病人中,共3847位病
人符合本研究之收案/排除條件。我們使用自然語言處理,萃取出住院初期之醫師紀錄及放射報告中之臨床文字紀錄,並且實驗了不同文字模型與機器學習演算法之組合,來建構中風功能復原後果的預測模型。實驗發現使用醫師紀錄時,操作特徵曲線下面積為0.782至0.805,而使用放射報告時,曲線下面積為0.718至0.730。使用醫師紀錄時,最好的組合為詞頻-倒文件頻加上羅吉斯迴歸,而使用放射報告時,最好之組合為基于轉換器的雙向編碼器表示技術加上支持向量機。這些基於純文字的機器學習預測模型並無法勝過傳統的風險模型,這些傳統模型的曲線下面積為0.811至0.841。然而,不管是以曲線下面積、重分類淨改善指標、或整合式
區辨改善指標來評估,臨床文字紀錄中的資訊的確可以增強傳統風險模型的預測效能。本研究之結論為,電子病歷中的非結構化文字經過自然語言處理後,不僅可以成為另類預測中風功能復原後果的工具,更可以增強傳統風險模型的預測效能。透過演算法來自動擷取並整合分析結構化與非結構化資料,將能提供醫師更好的決策支援。
想知道Party 2更多一定要看下面主題
Party 2的網路口碑排行榜
-
#1.Mario Party 2 瑪利歐派對2 Nintendo 64 編號:G3 | 露天拍賣
你在找的Mario Party 2 瑪利歐派對2│Nintendo 64│編號:G3就在露天拍賣,立即購買商品搶免運及優惠,還有許多相關商品提供瀏覽. 於 www.ruten.com.tw -
#2.High Party 2 (2006, CD) - Discogs
View credits, reviews, tracks and shop for the 2006 CD release of "High Party 2" on Discogs. 於 www.discogs.com -
#3.英語你我他-生活篇07 接人參加party(2) - 教育廣播電台 ...
第7集英語你我他-生活篇07 接人參加party(2). 1. 131. 分享. 英語. 邀訪來賓:播出日期:2005/09/13. 接人參加party(2). 心得筆記. 播放時間00:00. 心得筆記內容. 於 channelplus.ner.gov.tw -
#4.House Party 2 - 中文百科知識
《House Party 2》是由George Jackson 、 Doug McHenry 執導的一部喜劇類電影。基本信息Estudantes Devem Estar Loucos, Os.....Portugal Kid'n Play - Det vilda ... 於 www.easyatm.com.tw -
#5.MARIO PARTY 2 - Nintendo of Europe
Visit RULES LAND to learn the rules of the. MARIO PARTY 2 ADVENTURE BOARDS. It's a good idea to go here the first time you play. Visit MINI-GAME LAND to ... 於 cdn02.nintendo-europe.com -
#6.Mario Party Party: 2 - Giant Bomb
Mario Party Party: 2. It's another fifty turns of delight and wonderment and you're all invited! Join us as the Giant Bomb gang stares down the barrel of a ... 於 www.giantbomb.com -
#7.Skateboard Party 2安卓下載,安卓版APK | 免費下載
Skateboard Party 2 把滑板运动的所有乐趣带到你的移动设备,让你在8 个完全独特的地点尽享滑行。跳上你的滑板,学习新招式并提升你的滑板技巧并以超酷的连续技落地。 於 m.apkpure.com -
#8.購買Costume Party 2 - Awesomenauts Assemble! | Xbox
含有: Cap'n Vinnie & Seadog Spike Cluck Coco McFly Demon Skølldir Double-O Yuri Kage Genji Pimpy G Ravishing Raelynn Shaolin Ayla. 於 www.xbox.com -
#9.現貨全新Mario Party Super star party 2 - Nintendo 任天堂
喺Hong Kong,Hong Kong 買現貨全新Mario Party Super star party 2. 歡迎以舊換新PS/NS均可喺Nintendo 任天堂度買嘢,傾偈買嘢! 於 www.carousell.com.hk -
#10.What Made Mario Party 2 The Best Of A Bad Series - TheGamer
Mario Party has been regarded as one of the worst Mario titles ever: Nintendo did a better job with Mario Party 2. 於 www.thegamer.com -
#11.Play Fruit Party 2™ Slot Demo by Pragmatic Play
Play Fruit Party 2™ Videoslot by Pragmatic Play for free. Check out our amazing Casino Slots, Card Games, Table Games, Bingo and Live Casino Games. 於 www.pragmaticplay.com -
#12.Qipao Ladies Night Party 旗袍之夜 - 銅猴子
Watch out for our next theme party! gallery/2014/qipaoinside2. ... https://www.brassmonkeytaipei.com/2014/qipao-ladies-night-party-2-of-2-photos# ... 於 www.brassmonkeytaipei.com -
#13.House Party 2 - 華人百科
《House Party 2》是一部喜劇電影,導演為George Jackson Doug McHenry,編劇為Reginald Hudlin。外文名稱House Party 2編 劇Reginald Hudlin 類 型喜劇. 於 www.itsfun.com.tw -
#14.功夫Party 2
在Apple Music 聆聽The Party的《功夫Party 2》。串流〈功夫Party〉、〈歡樂不走開〉等歌曲。 於 music.apple.com -
#15.Skateboard Party 2 - Google Play 應用程式
Skateboard Party 2 把滑板运动的所有乐趣带到你的移动设备,让你在8 个完全独特的地点尽享滑行。跳上你的滑板,学习新招式并提升你的滑板技巧并以超 ... 於 play.google.com -
#16.眾神• 電Party 2-歌詞-董事長樂團|MyMusic 懂你想聽的
眾神• 電Party 2-歌詞-無歌詞-MyMusic懂你想聽的,打開APP立即開始聽歌. 於 www.mymusic.net.tw -
#17.開眼電影網
開眼 ﹥電影 ﹥家庭派對2 House Party 2. 家庭派對2 House Party 2. 當奇德的大學基金被無良的音樂出資人偷走,普瑞決定舉辦的睡衣派對作為解決之道。 於 app2.atmovies.com.tw -
#18.Spring Party 2/2/2020 6~9 PM | TAITA Silicon Valley
Dear TAITA friends,. This is a kind reminder that TAITA spring party is this coming Sunday (2/2) from 6pm to 9pm at China Stix. Due to recent concerns of ... 於 www.taita.org -
#19.Party 2 the Max
Party 2 The Max offers party attractions and rentals throughout Greater Fort Worth, TX. We are DFW's best choice for all-age activities. 於 www.party2themax.com -
#20.ONE PIECE PARTY 2卷| 航海王wiki
ONE PIECE PARTY航海王派對第2卷,日本於2016年7月4日發售。 標題為《DAVY BACK FIGHT》。 標題為《索隆走失》。 標題為《火焰永不消失》。 標題為《千陽號的客人》。 於 onepiece.fandom.com -
#21.【美麗PARTY二期】社區詳情|中古屋,租屋,房價查詢 - 591實價 ...
591為您提供:「美麗PARTY二期」位於高雄市鼓山區,屋齡15年,共146戶,實價登錄格局4房、坪數19.0~76.1坪,房價區間11.6~56.8萬/坪。更多美麗PARTY二期實價登錄、中古 ... 於 market.591.com.tw -
#22.瑪利歐派對2 (Mario Party 2)
瑪莉派對二Mario Party 2. 瑪莉歐派對2的隱藏要素 1.隱藏場地"庫巴區"(Bowser Land) 把另外五個場地全部至少玩過一次,就算輸了也沒關係 2. 於 mario.ngamer.net -
#23.Corpse Party 2: Dead Patient - Steam 社群
Corpse Party 2: Dead Patient. ... 不要再顯示Corpse Party 2: Dead Patient 的警告通知. 檢視頁面. 取消. 您的偏好設為在圖片可能含有敏感內容時提出警告。 於 steamcommunity.com -
#24.Mario Party 2 Cheats For Nintendo 64 - GameSpot
Mario Party 2 Cheats For Nintendo 64 · Access Bowser Land · Secret Minigames · Unlock 1P Coaster · Taunt · Win Coins when using a Mushroom · Unlock a new game type. 於 www.gamespot.com -
#25.功夫PARTY (二)
Listen to 功夫PARTY (二) on Spotify. The Party · Album · 1994 · 11 songs. 於 open.spotify.com -
#26.Third cat die-cut card 【BEER PARTY】 2 pieces - 卡片/明信片
Third cat die-cut card 【BEER PARTY】 2 pieces. 部分商品資訊是由Google 自動翻譯,內容不完全精確。 翻譯成中文-繁體顯示原文(日文). 於 www.pinkoi.com -
#27.取材熱門迪士尼頻道節目 - Ubisoft
《舞力全開》與迪士尼再度聯手,隆重獻上最厲害的舞蹈遊戲,結合風靡全球的迪士尼頻道節目和電影音樂供闔家熱舞:一切精彩盡在《Just Dance®: Disney Party 2》。 於 www.ubisoft.com -
#28.The Jackbox Party Pack 2
The Jackbox Party Pack 2. The sequel to the party game phenomenon The Jackbox Party Pack, featuring FIVE fresh, rib-tickling party games! 於 www.jackboxgames.com -
#29.Pixel Party 2 - Minecraft Maps
Pixel Party 2 is a party games map, that lets you choose between eight different fun and unique minigames. Select the amount of rounds you and ... 於 www.minecraftmaps.com -
#30.Mario Party 2
Mario Party 2 is the second main installment in the Mario Party series for the Nintendo 64. Mario and the gang are fighting for the rights for a new ... 於 www.mariowiki.com -
#31.House Party 2 movie review & film summary (1991) - Roger ...
"House Party 2" is a genial and good-humored sequel to the surprise 1990 hit, but not much more. It contains a lot of listenable music, ... 於 www.rogerebert.com -
#32.Tea Party II - 混得好《In the mix》 - 竅門設計事務所
Tea Party II · 混得好《 In the mix 》 · 我們創造的不只是茶席,更是台灣的茶文化觀點,我們尋找的不只是茶,而是台灣的味道。 於 www.studio-qiao.com -
#33.《HIStory3-那一天》獨家內容:HIStory Party 2 - LINE TV
《HIStory3-那一天》獨家內容:HIStory Party 2. BL館•已完結/ 共9 集. 4.9. 收藏. 分享. 參與演員. 宋偉恩|; 黃雋智|; 劉韋辰|; 張瀚元. 劇情簡介. 於 www.linetv.tw -
#34.Mario Party 2 - The Cutting Room Floor
Unused Graphics · Early Japanese Logo · Bomberman · Western Mario · Early Tumbleweed · Early Mushroom Genie Background · Japanese Mario Party Board ... 於 tcrf.net -
#35.House Party 2 Movie Review | Common Sense Media
Solid sequel has language, sex, and raunchy humor. Read Common Sense Media's House Party 2 review, age rating, and parents guide. 於 www.commonsensemedia.org -
#36.Projekt domu Party 2 (TIZ-956) - 127.37m² - Tooba.pl
Nowoczesny i elegancki dom parterowy z poddaszem użytkowym - projekt domu Party 2. Jego łączna powierzchnia wynosi nieco ponad 127 m². 於 www.tooba.pl -
#37.Ibiza Dance Party 2 - Universal Production Music
Uplifting dance, trance and techno anthems; a follow-up to CHAP AV155 "Ibiza Dance Party". 於 www.universalproductionmusic.com -
#38.文博會- Tea Party II 四季 - WORK - 當若科技藝術
Tea Party於文博會中展開一場文化混音的茶酒派對。2015 Tea Party 2以「多媒體互動」方式傳遞四季茶酒沙龍獨特之五感體驗,一場跨域表演,一種合拍默契。 於 if.plus -
#39.HIGH PARTY 2 專輯歌曲合輯(2006年發行 ... - 魔鏡歌詞
合輯(2006年發行) 歌詞集合台.港.大陸.新加坡.韓國.歐洲.美國最強舞曲陣容全力製作全台舞場DJ一至推薦16首舞霸中港台最火華語單曲全新高能量舞曲混音CD1+1單片 ... 於 mojim.com -
#40.Game Party II - Nintendo Wii - GameStop
Game Party 2 features new games like Bean Bags, Horseshoes, Lawn Darts, Puck Bowling and more. Each game, including classics like Skill Ball, Darts and Hoop ... 於 www.gamestop.com -
#41.disclosed to a third party 2 - Linguee | 中英词典(更多其他语言)
大量翻译例句关于""disclosed to a third party 2" – 英中词典以及8百万条中文译文例句搜索。 於 cn.linguee.com -
#42.The Pioneer Woman Garden Party 2-Piece Plastic Colander Set
Arrives by Fri, Mar 11 Buy The Pioneer Woman Garden Party 2-Piece Plastic Colander Set at Walmart.com. 於 www.walmart.com -
#43.Shed Party #2 - Young Studios
1 review for Shed Party #2. Rated 5 out of 5. Debby Lane – April 14, 2020. Your work is amazing!!! It is very difficult to choose a favourite. 於 ayoungstudios.com -
#44.KIM PETRAS ❤️ 在Twitter 上:"Malibu release party 2 night ...
Malibu release party 2 night 8pm pst on zoom space is limited so stay tuned for the code. 圖片. Aquaria 和其他1 人. 於 twitter.com -
#45.公主派對2 : 公主扮靚派對- PRINCESS PARTY 2 (DVD)_英語片
產品名稱: 公主派對2 : 公主扮靚派對- PRINCESS PARTY 2 (DVD). 介質/格式: DVD. 片裝數: 1. 國家地區: HK. 語種/配音: 英語/粵語配音. 字幕: 英/ 中/ 泰/ 印尼文. 於 cdwarehouse.com.hk -
#46.Clown Party 2 - Milk Cult | Shazam
聆聽Milk Cult 被Shazam 過4 次的Clown Party 2. 於 www.shazam.com -
#47.Get Snowboard Party 2 Lite - Microsoft Store
2016年2月14日 — HIGH DEFINITION Snowboard Party 2 includes next generation 3D graphics specially optimized for your mobile hardware to provide you with the ... 於 www.microsoft.com -
#48.DJ 小慌- 2021.99專屬(Party.2)
Stream DJ 小慌- 2021.99專屬(Party.2) by DJ小慌Remix on desktop and mobile. Play over 265 million tracks for free on SoundCloud. 於 soundcloud.com -
#49.Irastuoya Party 2 – LINE stickers
Stickers of a rabbit, a dog, a cat and a bear from Irastuoya dancing very happily at a party. 於 store.line.me -
#50.美麗Party二期:高雄市鼓山區文信路| 社區介紹 - 好房網
美麗Party二期位於高雄市鼓山區文信路。特色:衛浴開窗格局方正釋出稀少臨華榮公園明誠公園綠地周邊商圈生活機能極佳。周邊交通:R14捷運站旁,保值性佳臨文信路與明倫 ... 於 buy.housefun.com.tw -
#51.The Party 2 (1982) - IMDb
The Party 2: Directed by Claude Pinoteau. With Claude Brasseur, Brigitte Fossey, Sophie Marceau, Lambert Wilson. Vulnerable and impressionable teenage girl ... 於 www.imdb.com -
#52.high party 2 中文- 英語翻譯 - 查查在線詞典
high party 2中文:愛你啦…,點擊查查權威綫上辭典詳細解釋high party 2的中文翻譯,high party 2的發音,音標,用法和例句等。 於 tw.ichacha.net -
#53.Thursday Party 2 (首爾) - 餐廳/美食評論 - TripAdvisor
Thursday Party 2(首爾): 讀讀3則則關於Thursday Party 2客觀公正的美食評論,在Tripadvisor的5分滿分評等中得3分,在首爾的38127家餐廳中排第28220名。 於 www.tripadvisor.com.tw -
#54.Desert House Party 2 by Slim Aarons | FINEPRINT co
Desert House Party 2 · Artist: Slim Aarons · About this artwork · Customer Reviews · My escape to Palm Springs circa 1970. 於 fineprintco.com -
#55.Mario Party 2 - speedrun.com
Speedrunning leaderboards, resources, forums, and more! 於 www.speedrun.com -
#56.Corpse Party 2: Dead Patient – XSEED Games
Set five years after the events of Corpse Party: Blood Drive, Corpse Party 2: Dead Patient (which was released as Corpse Party 2: Dead Patient NEUES; ... 於 www.xseedgames.com -
#57.果然是大聲公-Divoom Voombox Party 2nd藍牙喇叭
如果您也有同樣的需求,不妨參考一下Divoom新推出的Voombox Party 2nd(以下簡稱Party 2)藍牙無線喇叭,看型號就知道,目前已經開發到第二代,查了 ... 於 review.u-audio.com.tw -
#58.遊戲Bullet Party 2在線。免費玩
遊戲Bullet Party 2 Bullet Party 2()在線。 於 cn.game-game.com -
#59.2019 HIStory Party II - 巧克科技新媒體
2019 HIStory Party II · 1. 此票區僅開放哈TV會員購買。 · 2. 此時段可購買哈TV+會員票區VIP 2,000元及哈TV+會員票區HAPPY區1,500元,採限區限量,售完為止 ... 於 chocolabs.kktix.cc -
#60.Mario Party 2 Prices Nintendo 64 - PriceCharting.com
Compare current and historic Mario Party 2 prices (Nintendo 64). Loose, Complete (CIB), and New prices updated daily. 於 www.pricecharting.com -
#61.o week 2022 white party 2 - Eventbrite
Eventbrite - UniPartiesMelb presents O WEEK 2022 WHITE PARTY 2 - Thursday, February 24, 2022 | Friday, February 25, 2022 at Brown Alley, ... 於 www.eventbrite.com.au -
#62.Party Of 2 - Online Shop
Partyof2, la linea di capi tecnici dal taglio fashion, per l'equitazione. 於 www.partyoftwo.it -
#63.Mario Party 2 - Wikipedia
Mario Party 2 is a party video game developed by Hudson Soft and published by Nintendo for the Nintendo 64. The second game in the Mario Party series, ... 於 en.wikipedia.org -
#64.美麗PARTY NO2 - 樂居
明倫路167號二樓. 房屋坪. 30.79 坪. 車位坪. 3.56 坪. 車位價. 80 萬. 總價. 787 萬. 23.39 萬/坪. 110年03月. 明倫路159號五樓. 房屋坪. 27.79 坪. 於 www.leju.com.tw -
#65.PAPERSELF刺青貼紙午茶兔女伶Rabbit Tea Party 2入| 誠品線上
PAPERSELF刺青貼紙午茶兔女伶Rabbit Tea Party 2入:,這個週末下午妳要不要跟我去喝杯下午茶?趁陽光正好、花朵正開,把所有甜甜的、美好的,都裝進生活裡吧! 於 www.eslite.com -
#66.Nickelodeon Block Party 2 Strategy Game - Nick UK
Your favourite Nickelodeon characters are back and better than ever in the free online action game, Block Party 2. Join the fun in this fun action game. 於 www.nick.co.uk -
#67.House Party 2 - Rotten Tomatoes
House Party 2 is a genial and good-humored sequel to the surprise 1990 hit, but not much more. January 1, 2000 | Rating: 2/4 | Full Review… 於 www.rottentomatoes.com -
#68.Play Bullet Party 2 on CrazyGames
Bullet Party 2 - Multiplayer FPS is a fast-paced multiplayer shooter game just like Bullet Force Online. Choose your game mode and show off your shooting ... 於 www.crazygames.com -
#69.RD4EA4 - Dance Dance Revolution: Hottest Party 2 - GameTDB
ID, RD4EA4. region, NTSC-U. type, Wii. languages, EN. title (EN), Dance Dance Revolution: Hottest Party 2. synopsis (EN), DanceDanceRevolution® celebrates ... 於 www.gametdb.com -
#70.High Party 2 專輯- Various Artists - KKBOX
Various Artists的專輯「High Party 2」在這裡,快打開KKBOX 盡情收聽。 於 www.kkbox.com -
#71.Mario Party 2 Nintendo 64 Video Games for sale | eBay
Get the best deals on Mario Party 2 Nintendo 64 Video Games and expand your gaming library with the largest online selection at eBay.com. 於 www.ebay.com -
#72.Corpse Party 2: Dead Patient - GOG.com
Corpse Party 2: Dead Patient ; Product details. 2019, Team GrisGris, ... ; System requirements. Windows 7 / 8 / 10, AMD FX™-6300, 4 GB RAM, Radeon RX 550 / ... 於 www.gog.com -
#73.House Party 2_百度百科
《House Party 2》是由George Jackson 、 Doug McHenry 執導的一部喜劇類電影。 於 baike.baidu.hk -
#74.Office party planning - British Council Learn English
Are the sentences true or false? Answer. 1. Ayako wants a baby grand piano for the Christmas party. 2. The baby grand piano will easily fit through ... 於 learnenglish.britishcouncil.org -
#75.Trivia Murder Party 2: Server never connects : r/jackboxgames
Trivia Murder Party 2: Server never connects. So I got Party Pack 6 this week for PS4. For whatever reason, I'm never able to connect to a server for TMP2. 於 www.reddit.com -
#76.Party 2 - Home | Facebook
Party 2 ; Night Area Partyroom. Party Entertainment Service. 441 people like this ; J2 party house. Sports & Recreation. 285 people like this ; Party Room - C- ... 於 www.facebook.com -
#77.《Mario Party Superstars》10月推100個Party小遊戲支援連線玩
任天堂於直播節目「Nintendo Direct」中正式宣佈,集合歷代《瑪利歐派對》系列當中的大富翁模式以及小遊戲的全新系列作品《瑪利歐派對超級巨星》 於 www.hk01.com -
#78.Nintendo 任天堂N64 卡帶MARIO PARTY2 瑪莉歐派對2
品相如圖.讀取正常.無其他配件. 裸卡賣.外觀有使用痕跡.保持原樣出貨購買Nintendo 任天堂N64 卡帶MARIO PARTY2 瑪莉歐派對2. 於 shopee.tw -
#79.Mario Party 2 的影片與精華 - Twitch
快來觀賞Twitch 上最新最精彩的《Mario Party 2》影片。註冊或登入來加入社群,並追隨您最愛的《Mario Party 2》實況主! 於 www.twitch.tv -
#80.Mario Party 2 - Video Games - Amazon.com
Mario party 2 is a multiplayer game with a story but the game is awesome for nintendo 64. Story: play as either Mario, Luigi, Yoshi, Peach, DK, and Wario in ... 於 www.amazon.com -
#81.Corpse Party 2: Dead Patient for PC Reviews - Metacritic
Metacritic Game Reviews, Corpse Party 2: Dead Patient for PC, High-schooler Ayame awakens from a coma in a seemingly abandoned hospital, ... 於 www.metacritic.com -
#82.Projekt domu Party 2 WAH1719 - koszt budowy - Extradom
Projekt domu z poddaszem Party 2 o pow. 130,21 m2 z obszernym garażem, z dachem dwuspadowym, z tarasem, sprawdź! 於 www.extradom.pl -
#83.The Party 2 - Prime Video
The Party 2. 6.21 小時47 分鐘198213+. Two years later Vic has turned into a ravishing fifteen year old girl whose love life takes up more of her time than ... 於 www.primevideo.com -
#84.mario party 2 - Best Buy
Shop for mario party 2 at Best Buy. Find low everyday prices and buy online for delivery or in-store pick-up. 於 www.bestbuy.com -
#85.在Steam 購買Corpse Party 2: Dead Patient 即可省下20%
Step into the latest episodic installment in the Corpse Party saga, and try your best to survive treatment. ... Corpse Party 2 doesn't suffer the same fate. 於 store.steampowered.com -
#86.Awesomenauts Assemble! – Costume Party 2 (英文版)
bfont color=orange※在購買本套裝包前若已購買單獨商品。fontbbrbr本內容將解鎖『Vinnie和Spike、Clunk、Coco、Skølldir、Yuri、Genji、Froggy G、Raelynn和Ayla』的 ... 於 store.playstation.com -
#87.Projekt domu Party 2 - 130.21 m2 - koszt budowy 190 tys. zł
Projekt domu Party 2 jest nową odsłoną popularnego projektu Party ⭐ z pomniejszoną bryłą ⭐ i nowoczesną stylistyką ⭐ Sprawdź teraz! 於 www.mgprojekt.com.pl -
#88.高雄市鼓山區文信路美麗Party二期最新實價登錄 - 永慶房屋
美麗Party二期位於高雄市鼓山區文信路,屋齡約16年,樓高15層,近1年平均實價登錄24萬/坪。永慶房仲網提供最新高雄市鼓山區美麗Party二期實價登錄、成交行情、 ... 於 community.yungching.com.tw -
#89.TEA PARTY II - HEY SHENG 黑生起司
TEA PARTY II ... The "mixing design" is developed from "mixing wine" and "brewing tea". Emerging action inertia and shaping context, the heights of utensils are ... 於 heysheng.com.tw -
#90.本日發布《Super Mario Party》免費更新,「雙陸遊戲」
本日4月27日(二)起發布《Super Mario Party》的免費更新,「雙陸遊戲(Mario Party)」、「70種小遊戲」、「Mario Party 2on2」能夠對應線上遊玩。 於 www.nintendo.com.hk -
#91.Dance Party (2019) #2 - Code.org
Dance Party - Events. Instructions. Immersive Reader. The when up ▽ pressed block lets you run code when you press the arrow buttons. 於 studio.code.org -
#92.「旗津滿月趴2」 Cijin Full Moon Party 2再度引爆高雄熱情
潘局長說,第二次「旗津滿月趴Cijin Full Moon Party」活動於上週開放1000名遊客免費報名,不到三天就額滿,觀光局將會每月持續辦理,歡迎全球各地的 ... 於 khh.travel -
#93.Projekt domu jednorodzinnego Party 2 (IP69)
Party 2 jest projektem domu jednorodzinnego, bez podpiwniczenia, energooszczędnym, parterowym z poddaszem, tanim w budowie, w stylu nowoczesnym, ... 於 wybieramprojekt.pl -
#94.新月餘暉映照荒野! 「MIDNIGHT PARTY」2月8日登場
「MIDNIGHT PARTY」2月8日登場. 23efgHJ_uil_Ool)_L'lii'_&K'.png. 只會轉出闇屬性角色的「MIDNIGHT PARTY」轉蛋活動,2021年02月08日中午再度現身! 於 www.monster-strike.com.tw