DeepMind paper的問題,透過圖書和論文來找解法和答案更準確安心。 我們找到下列股價、配息、目標價等股票新聞資訊
DeepMind paper的問題,我們搜遍了碩博士論文和台灣出版的書籍,推薦Brady, Chris,Tuyls, Karl,Omidshafiei, Shayegan寫的 AI for Sports 和Brady, Chris,Tuyls, Karl,Omidshafiei, Shayegan的 AI for Sports都 可以從中找到所需的評價。
另外網站Model Evaluation For Extreme Risks of AI - YouTube也說明:Get my A.I. + Business Newsletter (free):https://natural20.com/#ai #google # deepmind So today, DeepMind drops a new paper, an early warning ...
這兩本書分別來自 和所出版 。
國立陽明交通大學 資訊科學與工程研究所 吳毅成所指導 劉良甫的 應用 MuZero 演算法於 2048 遊戲 (2021),提出DeepMind paper關鍵因素是什麼,來自於MuZero、2048 遊戲、深度強化式學習、蒙地卡羅樹搜尋、隨機性環境、深度學習。
而第二篇論文國立臺灣大學 土木工程學研究所 張學孔所指導 王嵩容的 應用多智慧體強化學習優化自駕巴士營運之研究 (2021),提出因為有 自駕巴士、多智慧體強化學習、策略梯度演算法、車隊管理、社會成本的重點而找出了 DeepMind paper的解答。
最後網站DeepMind's new chatbot uses Google searches plus humans ...則補充:In a new non-peer-reviewed paper out today, the team unveils Sparrow, an AI chatbot that is trained on DeepMind's large language model ...
AI for Sports
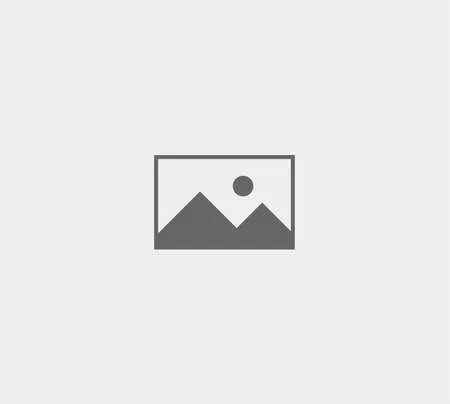
為了解決DeepMind paper 的問題,作者Brady, Chris,Tuyls, Karl,Omidshafiei, Shayegan 這樣論述:
Professor Chris Brady is currently the Chief Intelligence Officer at Sportsology, a US-based consultancy to elite sports organizations across the globe. Professor Brady has had a varied working life ranging from a line-worker at Chrysler in Detroit in his teens to managing a bookmaker’s shop, from a
land surveyor to a semi-professional footballer, from a naval officer to a management consultant. Prior to joining Sportsology he was most recently Professor of Management Studies at Salford University where he founded the Centre for Sports Business which focussed on the production of high quality
research with a particular emphasis on statistical analytics and future trend analysis within the global sports industry.Karl Tuyls (FBCS) is a team lead at DeepMind (Paris, France), an honorary professor of Computer Science at the University of Liverpool, UK, and a Guest Professor at the University
of Leuven, Belgium. Previously, he held academic positions at the Vrije Universiteit Brussel, Hasselt University, Eindhoven University of Technology, and Maastricht University. Prof. Tuyls has received several awards with his research, amongst which: the Information Technology prize 2000 in Belgium
, best demo award at AAMAS’12, winner of various Robocup@Work competitions (’13, ’14), and he was a co-author of the runner-up best paper award at ICML’18. Furthermore, his research has received substantial attention from national and international press and media, most recently his work on Sports A
nalytics featured in Wired UK. He is a fellow of the British Computer Society (BCS), is on the editorial board of the Journal of Autonomous Agents and Multi-Agent Systems, and is editor-in-chief of the Springer briefs series on Intelligent Systems. Prof. Tuyls is also an emeritus member of the board
of directors of the International Foundation for Autonomous Agents and Multiagent Systems.Shayegan Omidshafiei is a senior research scientist in DeepMind’s Game Theory team, where he also co-leads DeepMind’s Sports Analytics effort. His research interests include multiagent systems, reinforcement l
earning, robotics, and control systems. He previously received his Ph.D. at the Laboratory for Information and Decision Systems (LIDS) and Aerospace Controls Laboratory (ACL) at MIT. He received a B.A.Sc. degree from the University of Toronto in 2012, and an S.M. degree in Aeronautics and Astronauti
cs from MIT in 2015. He is co-inventor of 5 patents filed with the United States Patent Office.
應用 MuZero 演算法於 2048 遊戲
為了解決DeepMind paper 的問題,作者劉良甫 這樣論述:
本篇論文基於 Google DeepMind 團隊於 2020 所發表的深度強化式學習演算法 MuZero,提出一個適用於隨機性遊戲環境的版本。除了讓模型學會當發生某個隨機事件時,環境會如何變化外,也學會在各個環境狀況下,各種隨機事件的發生機率,進而使模型能在執行蒙地卡羅樹搜尋 (Monte Carlo Tree Search) 時不依靠環境模擬器。我們選擇 2048 遊戲作為實驗環境,並研究相關超參數 (Hyper-parameter) 對於此環境訓練的影響。如:類神經網路骨幹架構、類神經網路深度及寬度、蒙地卡羅樹搜尋的 Simulation count、動作決策 (Action Poli
cy) 的 Softmax temperature、N-steps value 與 Discount,以及 Optimizer 的 Learning rate ,試圖找出相對適合 2048 遊戲的超參數設定。另外,本論文也基於 2048 遊戲的特性,試驗三種可能增進深度強化式學習 (Deep Reinforcement Learning) 的技巧,包含修改深度類神經網路的輸入資料,來讓模型更容易辨識;多階段訓練 (Multi-Stages),使得訓練資料更加平均;盤面重啟與初始化策略,方便讓模型更快突破遊戲當前的困境。最終,在經過 1,000 GPU hours 訓練後,於驗證 200 場的情
況下達到平均分數 327,937 分,各 tile 比例為:32,768-tile 13.5%、16,384-tile 73.5%、8,192-tile 91%。
AI for Sports
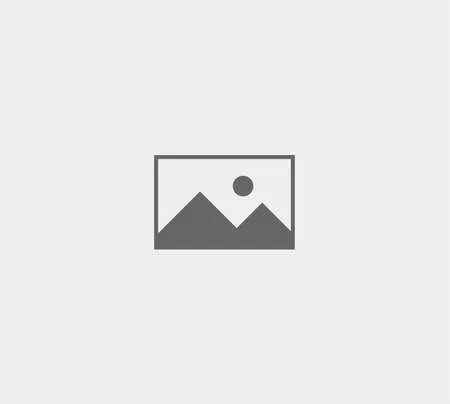
為了解決DeepMind paper 的問題,作者Brady, Chris,Tuyls, Karl,Omidshafiei, Shayegan 這樣論述:
Professor Chris Brady is currently the Chief Intelligence Officer at Sportsology, a US-based consultancy to elite sports organizations across the globe. Professor Brady has had a varied working life ranging from a line-worker at Chrysler in Detroit in his teens to managing a bookmaker’s shop, from a
land surveyor to a semi-professional footballer, from a naval officer to a management consultant. Prior to joining Sportsology he was most recently Professor of Management Studies at Salford University where he founded the Centre for Sports Business which focussed on the production of high quality
research with a particular emphasis on statistical analytics and future trend analysis within the global sports industry.Karl Tuyls (FBCS) is a team lead at DeepMind (Paris, France), an honorary professor of Computer Science at the University of Liverpool, UK, and a Guest Professor at the University
of Leuven, Belgium. Previously, he held academic positions at the Vrije Universiteit Brussel, Hasselt University, Eindhoven University of Technology, and Maastricht University. Prof. Tuyls has received several awards with his research, amongst which: the Information Technology prize 2000 in Belgium
, best demo award at AAMAS’12, winner of various Robocup@Work competitions (’13, ’14), and he was a co-author of the runner-up best paper award at ICML’18. Furthermore, his research has received substantial attention from national and international press and media, most recently his work on Sports A
nalytics featured in Wired UK. He is a fellow of the British Computer Society (BCS), is on the editorial board of the Journal of Autonomous Agents and Multi-Agent Systems, and is editor-in-chief of the Springer briefs series on Intelligent Systems. Prof. Tuyls is also an emeritus member of the board
of directors of the International Foundation for Autonomous Agents and Multiagent Systems.Shayegan Omidshafiei is a senior research scientist in DeepMind’s Game Theory team, where he also co-leads DeepMind’s Sports Analytics effort. His research interests include multiagent systems, reinforcement l
earning, robotics, and control systems. He previously received his Ph.D. at the Laboratory for Information and Decision Systems (LIDS) and Aerospace Controls Laboratory (ACL) at MIT. He received a B.A.Sc. degree from the University of Toronto in 2012, and an S.M. degree in Aeronautics and Astronauti
cs from MIT in 2015. He is co-inventor of 5 patents filed with the United States Patent Office.
應用多智慧體強化學習優化自駕巴士營運之研究
為了解決DeepMind paper 的問題,作者王嵩容 這樣論述:
自駕巴士過去五年在全世界超過七十幾個城市推廣測試,先進的無人駕駛技術得以免除駕駛人力成本、降低交通事故,有很大的潛力為公共運輸系統帶來革命性的變革。本研究的目標是發展一個自駕巴士車隊管理模式,讓自駕巴士能夠有效率的運作,同時比較自駕巴士的相對優勢。考量自駕巴士本質上是去中央指派的獨立運作智慧體,這些智慧體所面對的是旅客隨機到達以及部份環境資訊,在沒有人為干涉的狀況下學習作出決策。本研究基於自駕巴士在單一路動態服務特性而發展了一個「多智慧體強化學習」(MARL)車隊派遣方法,結合最先進的策略梯度(PG)演算法,來解決複雜且動態的自駕巴士車隊派遣最佳化問題。本研究同時發展一個自駕巴士往返路線的智
慧體動態模擬平台,用來訓練和評估此強化學習派遣演算法的績效。模擬結果顯示,本研究所發展的自駕巴士強化學習派遣,在相對較低乘客需求狀況,比較現行固定派遣公車具有降低車隊規模和減少乘客等待時間的優勢。本研究也同時探討自駕巴士強化學習派遣在一條往返公車路線上的社會總成本,包括業者營運成本以及乘客等待時間成本與乘客車內時間成本。研究結果顯示自駕巴士人工智慧派遣在社會成本最佳化狀況下,較現行固定班表普通公車具有明顯的成本優勢。研究成果可以作為單一路廊自駕巴士派遣、優化以及未來自駕巴士系統發展的基礎。
想知道DeepMind paper更多一定要看下面主題
DeepMind paper的網路口碑排行榜
-
#1.Mathematicians use DeepMind AI to create new methods in ...
As a co-author of the paper, he applied the power of Deep Mind's AI processes to explore conjectures in his field of speciality, ... 於 www.sydney.edu.au -
#2.Industry leaders warn 'AI poses risk of extinction' - Mint
e-paper Subscribe ... OpenAI CEO Sam Altman, Google DeepMind chief executive Demis Hassabis, and Anthropic's chief executive Dario Amodei. 於 www.livemint.com -
#3.Model Evaluation For Extreme Risks of AI - YouTube
Get my A.I. + Business Newsletter (free):https://natural20.com/#ai #google # deepmind So today, DeepMind drops a new paper, an early warning ... 於 www.youtube.com -
#4.DeepMind's new chatbot uses Google searches plus humans ...
In a new non-peer-reviewed paper out today, the team unveils Sparrow, an AI chatbot that is trained on DeepMind's large language model ... 於 www.technologyreview.com -
#5.DeepMind AlphaTensor: The delicate balance between ...
Human intuition and computing power. In an essay not so long ago, I argued that the technology that we consider as artificial intelligence today ... 於 bdtechtalks.com -
#6.help understanding deep Q learning algorithm from deep ...
I'm studying this paper https://storage.googleapis.com/deepmind-media/dqn/DQNNaturePaper.pdf, and I have a question about proposed ... 於 datascience.stackexchange.com -
#7.DeepMind's quest for AGI may not be successful, say AI ...
Computer scientists are questioning whether DeepMind will ever be able ... DeepMind's “Reward is enough” paper was co-authored by DeepMind ... 於 www.cnbc.com -
#8.New research shows what AI can teach us about the human ...
Papers from Google's DeepMind show what we can learn from AI. ... The first paper explores how recent advances in “reinforcement learning” ... 於 www.vox.com -
#9.Human-level control through deep reinforcement learning
The theory of reinforcement learning provides a normative account, deeply rooted in psychological and neuroscientific perspectives on animal ... 於 www.nature.com -
#10.Rainbow: Combining Improvements in Deep Reinforcement ...
This paper examines six extensions to the DQN algorithm and empirically studies their combination. Our experiments show that the combination ... 於 aaai.org -
#11.No, AI probably won't kill us all - Asia Times
In March, Microsoft researchers published a paper claiming GPT-4 showed ... recently met with the leadership of Google DeepMind and OpenAI. 於 asiatimes.com -
#12.No, AI probably won't kill us all – and there's more to this fear ...
In March, Microsoft researchers published a paper claiming GPT-4 showed ... recently met with the leadership of Google DeepMind and OpenAI. 於 newssgoog.site -
#13.ANALYSING MATHEMATICAL REASONING ABILITIES
Published as a conference paper at ICLR 2019. ANALYSING MATHEMATICAL REASONING ABILITIES. OF NEURAL MODELS. David Saxton. DeepMind [email protected]. 於 openreview.net -
#14.Training Compute-Optimal Large Language Models - arXiv
Download a PDF of the paper titled Training Compute-Optimal Large Language Models, by Jordan Hoffmann and 21 other authors. 於 arxiv.org -
#15.UK to work with AI firms to ensure society benefits from the ...
British PM Rishi Sunak and the tech leaders - OpenAI's Sam Altman, Google DeepMind's Demis Hassabis and Anthropic's Dario Amodei - discussed ... 於 economictimes.indiatimes.com -
#16.DeepMind Paper Provides a Mathematically Precise ... - Medium
DeepMind Paper Provides a Mathematically Precise Overview of Transformer Architectures and Algorithms · Written by Synced · More from Synced and ... 於 medium.com -
#17.First Open Source Implementation of DeepMind's AlphaTensor
The algorithm is not deeply explained in the AlphaTensor paper, since it is based on several DeepMind's previous papers which are just cited and ... 於 www.kdnuggets.com -
#18.AI risk; Nvidia, the chip king; cyberattacks and kissing++ #424
Risky business. Major AI companies are coming out with recommendations on how to manage AI risks, the last of which was Google Deepmind's paper ... 於 www.exponentialview.co -
#19.DeepMind AGI paper adds urgency to ethical AI | VentureBeat
DeepMind expects reinforcement learning will lead to replicating human cognitive abilities, achieving artificial general intelligence (AGI). 於 venturebeat.com -
#20.Research
Our pioneering research includes Deep Learning, Reinforcement Learning, Theory & Foundations, Neuroscience, Unsupervised Learning & Generative Models, ... 於 www.deepmind.com -
#21.Junhyuk Oh
Jun 2018: I joined DeepMind as a research scientist. ... International Conference on Learning Representations (ICLR), 2023. Paper 於 junhyuk.com -
#22.UAlberta expertise brings DeepMind lab to Edmonton | Folio
One of the world's leading AI research companies, DeepMind, will open its first ... DeepStack paper published earlier this year in Science. 於 www.ualberta.ca -
#23.AlphaFold Protein Structure Database
AlphaFold is an AI system developed by DeepMind that predicts a protein's 3D structure from ... If you use this resource, please cite the following papers:. 於 alphafold.ebi.ac.uk -
#24.DeepMind Shows How A.I. Can Impact Software Development
If you're curious about how AlphaCode can generate “competition-level” code, check out DeepMind's paper detailing how it works. Competitive ... 於 www.dice.com -
#25.DeepMind feuds with Russian scientists over quantum AI ...
DeepMind, an Alphabet research company based in London, published a fascinating research paper last year wherein it claimed to have solved ... 於 thenextweb.com -
#26.Google Deepmind Researcher Co-Authors Paper ... - Slashdot
... to a new paper [from researchers at the University of Oxford and affiliated with Google DeepMind], but we don't have to wait to rein in. 於 slashdot.org -
#27.The Only Way to Deal With the Threat From AI? Shut It Down
The other leading AI lab, DeepMind, has no plan at all. An aside: None of this danger depends on whether or not AIs are or can be conscious; ... 於 time.com -
#28.Google DeepMind references a paper published by SBEL ...
Google DeepMind references a paper published by SBEL researchers and colleagues to motivate recent acquisition of MuJoCo for simulation in ... 於 sbel.wisc.edu -
#29.Google's Deep Mind Gives AI a Memory Boost That Lets It ...
Google's Deep Mind AI lab is giving deep learning software external ... for the Google DeepMind paper that describes development of this ... 於 spectrum.ieee.org -
#30.Reinforcement learning and e.g. deep kernel ... - Julia Discourse
I was scanning the paper, i.e. Reward is enough - ScienceDirect Reward is ... so while I'm not sure I believe Google's Deepmind paper, ... 於 discourse.julialang.org -
#31.DeepMind's AI is teaching itself parkour, and the ... - The Verge
Case in point is a new paper from Google's AI subsidiary DeepMind titled “Emergence of Locomotion Behaviours in Rich Environments. 於 www.theverge.com -
#32.Search for deepmind/deepmind-research - Papers With Code
We provide experimental results on the ColorMnist and CelebA benchmark datasets that quantify the properties of the learned representations and compare the ... 於 paperswithcode.com -
#33.Google Deepmind Scientist Warns AI Existential Catastrophe ...
A paper co-authored by a senior scientist at Google's artificial intelligence (AI) research laboratory DeepMind has concluded that advanced ... 於 www.iflscience.com -
#34.PM meeting with leading CEOs in AI: 24 May 2023 - GOV.UK
Prime Minister Rishi Sunak met the CEOs of OpenAI, Google DeepMind, ... the risks of the technology, as outlined in our AI White Paper. 於 www.gov.uk -
#35.Alpha Signal | The newsletter for AI professionals
Get a weekly summary of the top research papers, repos, and tweets identified ... Implementations and illustrative code to accompany DeepMind publications. 於 alphasignal.ai -
#36.Deepmind Ada brings foundation models to reinforcement ...
In the paper, the company trained a model with 265 million parameters and showed that 500 million parameters are possible with the method. 於 the-decoder.com -
#37.Illustrating Reinforcement Learning from Human Feedback ...
DeepMind used their 280 billion parameter model Gopher. ... In multiple papers from OpenAI, Anthropic, and DeepMind, this penalty has been ... 於 huggingface.co -
#38.Pause Giant AI Experiments: An Open Letter
Victoria Krakovna, DeepMind, Research Scientist, co-founder of Future of Life Institute. Emilia Javorsky, Physician-Scientist & Director, ... 於 futureoflife.org -
#39.Yi Yang's Homepage - DeepMind
Paper • Dataset. Occlusion Aware Unsupervised Learning of Optical Flow Yang Wang, Yi Yang, Zhenheng Yang, Liang Zhao, Peng Wang, Wei Xu CVPR 2018. 於 yangyi02.github.io -
#40.CLIP: Connecting text and images - OpenAI
Finally, CLIP is part of a group of papers revisiting learning visual representations from natural language supervision in the past year. 於 openai.com -
#41.DeepMind Researchers Develop 'Gato': A Multi-Modal, Multi ...
Home Tech News AI Paper Summary DeepMind Researchers Develop 'Gato': A Multi-Modal, Multi-Task, Multi-Embodiment AI Generalist Policy Tool... 於 www.marktechpost.com -
#42.Google DeepMind paper about AI's catastrophic risk - Reddit
So Google DeepMind as well as OpenAI, Anthropic and multiple universities and centers than study existential risks have put together a paper ... 於 www.reddit.com -
#43.Magenta
A research project exploring the role of machine learning in the process of creating art and music. 於 magenta.tensorflow.org -
#44.DeepMind Simulates Matter on the Nanoscale With Artificial ...
In a paper published in the scientific journal Science, DeepMind demonstrates how neural networks can be used to describe electron interactions ... 於 scitechdaily.com -
#45.How DeepMind discovered new ways of multiplying matrices ...
Last month, DeepMind published a paper where they presented AlphaTensor, an AI algorithm able to find faster ways of doing one of the most ... 於 towardsdatascience.com -
#46.Andrew Lampinen, DeepMind: On symbolic behavior, mental ...
Andrew Lampinen, DeepMind: On symbolic behavior, mental time travel ... we talked about a bunch of the symbolic behavior paper was the value ... 於 generallyintelligent.com -
#47.[PDF] DeepMind Lab - Semantic Scholar
DeepMind Lab is a first-person 3D game platform designed for research ... Figures and Tables; 195 Citations; 18 References; Related Papers ... 於 www.semanticscholar.org -
#48.Human-Timescale Adaptation in an Open-Ended Task Space
See figure E.1 in the paper for a comparison between agent and human scores on these two tasks. 於 sites.google.com -
#49.DeepMind unveils how it solved a 50-year-old scientific ...
DeepMind, the London-based artificial intelligence company, ... In its Nature paper, DeepMind wrote that AlphaFold 2 has already helped ... 於 fortune.com -
#50.DeepMind releases trio of papers on large language models
On Wednesday, DeepMind—the Alphabet–owned AI research company—published not one, not two, but three research papers about this year's most hotly ... 於 www.emergingtechbrew.com -
#51.Imagen: Text-to-Image Diffusion Models
There are several ethical challenges facing text-to-image research broadly. We offer a more detailed exploration of these challenges in our paper and offer a ... 於 imagen.research.google -
#52.Top 8 research papers by DeepMind in 2022 (till date)
Top 8 research papers by DeepMind in 2022 (till date) · An empirical analysis of compute-optimal large language model training · Restoring and ... 於 analyticsindiamag.com -
#53.Andrej Karpathy
... and finally at DeepMind in 2015 working on the deep reinforcement learning team. ... arxiv-sanity tames the overwhelming flood of papers on Arxiv. 於 karpathy.ai -
#54.DeepMind and Oxford University researchers on how to ...
In a moment where society is collectively reckoning with just how deep the roots of racism reach, a new paper from researchers at DeepMind ... 於 www.engadget.com -
#55.Google DeepMind - Wikipedia
Google DeepMind, formerly DeepMind Technologies, is a British artificial intelligence research laboratory which serves as a subsidiary of Google. 於 en.wikipedia.org -
#56.RLHF: Reinforcement Learning from Human Feedback
In collaboration with DeepMind's safety team, we've developed an algorithm ... In the InstructGPT paper, LLMSFT is represented as πSFT. 於 huyenchip.com -
#57.Google Deepmind Mastering Go Research Paper - SlideShare
Google Deepmind Mastering Go Research Paper. Mastering the Game of Go with Deep Neural Networks and Tree Search David Silver1 *, Aja Huang1 ... 於 www.slideshare.net -
#58.DeepMind's generalist AI, Gato: A non-technical explainer
SUMMARY DeepMind's recent paper, A Generalist Agent, catalyzed a wave of discourse regarding the speed at which current artificial ... 於 forum.effectivealtruism.org -
#59.AI Pub on Twitter: "DeepMind announces AdA: - Twitter
Episode 1 of "Deep Papers", a 4-part series interviewing AI scientists on their ... DeepMind announces AdA: - an agent RL pre-trained, ... 於 twitter.com -
#60.What is Artificial Intelligence (AI) ? | IBM
In this paper, Turing, often referred to as the "father of computer science", ... 2016: DeepMind's AlphaGo program, powered by a deep neural network, ... 於 www.ibm.com -
#61.DeepMind Paper Provides a Mathematically Precise ... - Synced
DeepMind Paper Provides a Mathematically Precise Overview of Transformer Architectures and Algorithms · Share this: · Like this: · About Synced. 於 syncedreview.com -
#62.LaMDA: our breakthrough conversation technology
Copy link. EliCollins1637. Eli Collins. VP, Product Management. Z. Zoubin Ghahramani. Vice President, Google DeepMind. 於 blog.google -
#63.Google Quantum AI
Google Quantum AI is advancing the state of the art in quantum computing and developing the hardware and software tools to operate beyond classical ... 於 quantumai.google -
#64.Two New Papers By Deepmind Exemplify How Artificial ...
In late 2021 and just now in October 2022, Deepmind released two papers describing new ways in which AI can help to develop mathematical ... 於 pub.towardsai.net -
#65.Deep Q-Learning for Atari Breakout - Keras
The Deepmind paper trained for "a total of 50 million frames (that is, around 38 days of game experience in total)". 於 keras.io -
#66.Must read: the 100 most cited AI papers in 2022 - Zeta Alpha
A detailed analysis of the 100 most cited papers per year, for 2022, ... The United States and Google still dominate, and DeepMind has had a ... 於 www.zeta-alpha.com -
#67.AI may destroy humanity, DeepMind scientists claim in co ...
A new paper co-authored by the University of Oxford and Google DeepMind researchers published last month in the peer-reviewed AI Magazine argues ... 於 interestingengineering.com -
#68.AI Poses Risks At Par With Pandemics & Nuclear Wars, Claim ...
AI Poses Risks At Par With Pandemics & Nuclear Wars, Claim DeepMind, ... despite having published an AI white paper two months earlier, ... 於 news.abplive.com -
#69.London Lab Advances Use of A.I. in Health Care, but Raises ...
In a paper published on Wednesday in the science journal Nature, researchers from DeepMind, a London artificial intelligence lab owned by ... 於 www.nytimes.com -
#70.Simple Alpha Zero - web.stanford.edu
The methods are fairly simple compared to previous papers by DeepMind, ... ideas from the AlphaGo Zero paper and understand them concretely through code. 於 web.stanford.edu -
#71.Response to DeepMind | SpringerLink
It is to a newspaper article that quotes Mustafa Suleyman who makes clear that the app may well integrate AI or machine learning approaches in ... 於 link.springer.com -
#72.Google Deepmind Researcher Co-Authors Paper ... - VICE
The paper, published last month in the peer-reviewed AI Magazine, is a fascinating one that tries to think through how artificial intelligence ... 於 www.vice.com -
#73.DeepMind researchers provide theoretical analysis on ...
DeepMind researchers published a paper last week, titled 'Degenerate Feedback Loops in Recommender Systems'. In the paper, researchers ... 於 hub.packtpub.com -
#74.DeepMind simulates matter on the nanoscale with AI
In a paper published by Science, DeepMind demonstrates how neural networks can improve approximation of the Density Functional (a method used to ... 於 scienmag.com -
#75.Humans beat DeepMind AI in creating algorithm to multiply ...
DeepMind's paper revealed a new method for multiplying two … five-by-five matrices in just 96 multiplications, two fewer than the previous ... 於 www.newscientist.com -
#76.What is ChatGPT, DALL-E, and generative AI? | McKinsey
DeepMind is a subsidiary of Alphabet, the parent company of Google, ... a “solid A-” essay comparing theories of nationalism from Benedict ... 於 www.mckinsey.com -
#77.DeepMind's Nature Paper and Earlier Related Work - IDSIA
The abstract of DeepMind's paper [2] on learning to play video games claims: "While reinforcement learning agents have achieved some ... 於 people.idsia.ch -
#78.Deep Reinforcement Learning Hands-On: Apply modern RL ...
Since DeepMind published its paper on the deep Q-network (DQN) model (https://deepmind.com/research/publications/playing-atari-deepreinforcement-learning) ... 於 books.google.com.tw -
#79.DeepMind's 'Gato' is mediocre, so why did they build it? - ZDNET
DeepMind's program is a generalist, to test the notion that over time, ... paper, "A Generalist Agent," posted on the Arxiv preprint server. 於 www.zdnet.com -
#80.DeepMind Flamingo: A Visual Language Model for Few-Shot ...
The tables and figures are taken from the original Flamingo paper with a few minor changes to the image captions and descriptions.. What is ... 於 wandb.ai -
#81.Summary of the AlphaGo paper
It “intuitively” evaluates board positions and potential moves through deep neural networks which is the method DeepMind had used to build their ... 於 becominghuman.ai -
#82.DeepMind Sparrow Dialogue model: Prompt & rules
Articles and papers (most recent at top). AI reports by Dr Thompson Cited: NBER AI report for US Government · The ChatGPT Prompt Book · Roadmap: ... 於 lifearchitect.ai -
#83.DeepMind Research - GitHub
Along with publishing papers to accompany research conducted at DeepMind, we release open-source environments, data sets, and code to enable the broader ... 於 github.com -
#84.The 21 smartest AI scientists working at Google DeepMind
Google acquired UK artificial intelligence startup DeepMind for ... A page on the DeepMind website details all of these academics papers and ... 於 www.businessinsider.com -
#85.DeepMind's Barkour: A New Benchmark for Quadrupedal ...
Google's DeepMind recently released a research paper outlining a potential benchmarking system called “Barkour” to quantify the performance ... 於 www.globalvillagespace.com -
#86.DeepMind's AlphaCode AI writes code at a competitive level
DeepMind's paper throws a little friendly shade on the competition in describing why it is going after the domain of competitive coding:. 於 techcrunch.com -
#87.Caution when interpreting Deepmind's In-context RL paper
Lots of people I know have had pretty strong reactions to the recent Deepmind paper, which claims to have gotten a transformer to learn an ... 於 www.lesswrong.com -
#88.Google and Oxford Scientists Publish Paper Claiming AI Will ...
Researchers at Google Deepmind and the University of Oxford have concluded that it is now "likely" superintelligent AI will be the end of humanity. 於 futurism.com -
#89.Google DeepMind introduces Barkour, a benchmark for ...
... DeepMind (which recently absorbed a large chunk of Alphabet's beleaguered Everyday Robots team) just issued a research paper outlining a ... 於 ca.style.yahoo.com -
#90.My quick notes on DeepMind's GATO paper - LinkedIn
DeepMind "A Generalist Agent" GATO paper: https://arxiv.org/pdf/2205. 於 www.linkedin.com -
#91.Implementing Responsible Data Enrichment Practices at an AI ...
This case study documents an effort to put that paper's recommendations into practice at one AI developer: DeepMind, a PAI Partner. 於 partnershiponai.org -
#92.InstaDeep-DeepMind Paper accepted at NeurIPS 2020 ...
InstaDeep-DeepMind Paper accepted at NeurIPS 2020 Workshop. AlphaNPI-X brings more structure in robotics skills learning to improve ... 於 www.instadeep.com -
#93.DeepMind's AI Trained For 5 Years... But Why? - YouTube
Check out Lambda here and sign up for their GPU Cloud: https://lambdalabs.com/ papers The paper ... DeepMind's AI Trained For 5 Years. 於 www.youtube.com -
#94.Deepmind's new model Gato is amazing! - Louis Bouchard
And as per Deepmind, this is not only a transformer but also an agent. This is what happens when you mix ... Image from Deepmind's paper. 於 www.louisbouchard.ai -
#95.DeepMind's New AI Looked At 1000000000 Images! - YouTube
DeepMind's New AI Looked At 1,000,000,000 Images! Two Minute Papers. 於 www.youtube.com -
#96.Playing Atari with Deep Reinforcement Learning
{vlad,koray,david,alex.graves,ioannis,daan,martin.riedmiller} @ deepmind.com ... This paper demonstrates that a convolutional neural network can overcome ... 於 www.cs.toronto.edu -
#97.What is the Deepmind Nowcasting paper all about?
Deepmind have now published a paper that uses classical radar data and deep learning to improve short-term weather forecasts. This work was produced in ... 於 dramsch.net -
#98.Explained Simply: How DeepMind taught AI to play video games
Space Invaders! Today we're going to take that original research paper and break it down paragraph-by-paragraph. This will make it more ... 於 www.freecodecamp.org